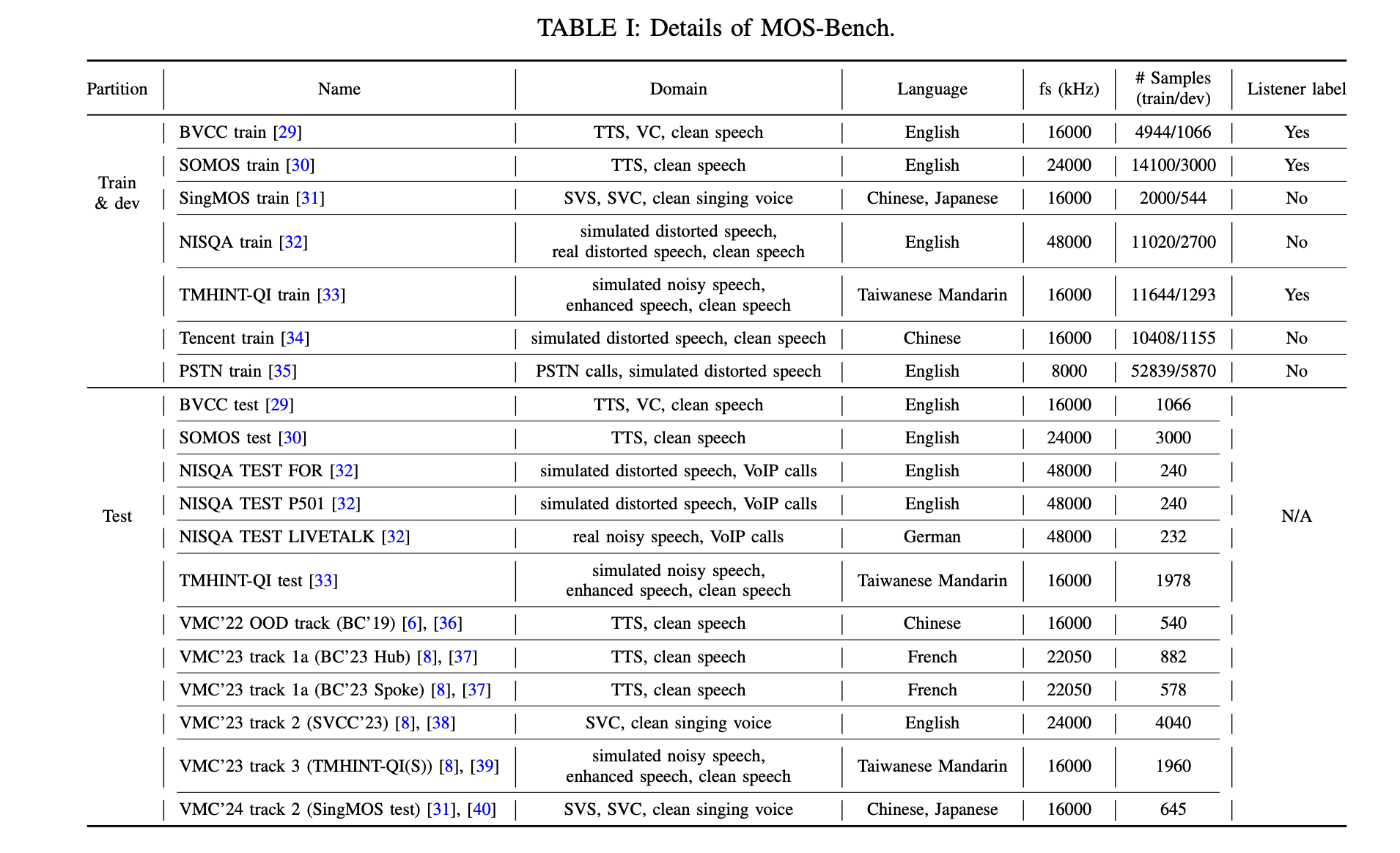
Understanding the Challenge in Speech Quality Assessment
A major issue in Subjective Speech Quality Assessment (SSQA) is helping models perform well across different speech types. Many existing models struggle when faced with new data because they are trained on specific types, limiting their real-world applications, like automated evaluations for Text-to-Speech (TTS) and Voice Conversion (VC) systems.
Current Approaches and Their Limitations
There are two main methods in SSQA:
- Reference-based models: These compare speech samples to a standard reference.
- Model-based methods: These use Deep Neural Networks (DNNs) that learn from human-annotated data.
While model-based methods can capture human perception better, they face significant challenges:
- Generalization Issues: Models often fail when tested with new data.
- Dataset Bias: Models may become too reliant on the training data, making them less effective elsewhere.
- High Computational Costs: Complex models can be too resource-intensive for real-time use.
Introducing MOS-Bench and SHEET
To overcome these challenges, researchers have developed MOS-Bench, a collection of datasets that includes various speech types, languages, and sampling frequencies. Coupled with SHEET, a toolkit for training and testing SSQA models, these resources provide a systematic way to evaluate model performance and improve generalization.
Key Features of MOS-Bench and SHEET
- Multi-Dataset Approach: MOS-Bench combines data from different sources to expose models to various conditions.
- New Performance Metrics: A new scoring method offers a comprehensive assessment of model performance.
- Robust Training Workflows: SHEET streamlines data processing, training, and evaluation, enhancing model reliability.
Benefits of Using MOS-Bench and SHEET
By using these tools, models can generalize better across different types of data, achieving high accuracy even with unfamiliar datasets. This development is a significant step forward in automated speech quality assessment.
Next Steps for Researchers and Companies
With the resources provided by MOS-Bench and SHEET, researchers can create more adaptable SSQA models that perform well across various speech types. This advancement opens doors for real-world applications, making SSQA more effective.
Explore the Paper: All credit goes to the researchers behind this project. Stay updated by following us on Twitter, joining our Telegram Channel, and being part of our LinkedIn Group. If you appreciate our work, consider subscribing to our newsletter and joining our 55k+ ML SubReddit.
Leverage AI for Your Business
To stay competitive, consider using MOS-Bench for training and evaluating SSQA models:
- Identify Automation Opportunities: Find key areas in customer interactions that AI can enhance.
- Define KPIs: Ensure your AI initiatives have measurable impacts.
- Select an AI Solution: Choose tools that meet your specific needs.
- Implement Gradually: Start with pilot projects, gather data, and expand wisely.
For advice on AI KPI management, contact us at hello@itinai.com. For ongoing insights, follow us on Telegram or Twitter.
Discover how AI can transform your sales processes and customer engagement by exploring solutions at itinai.com.