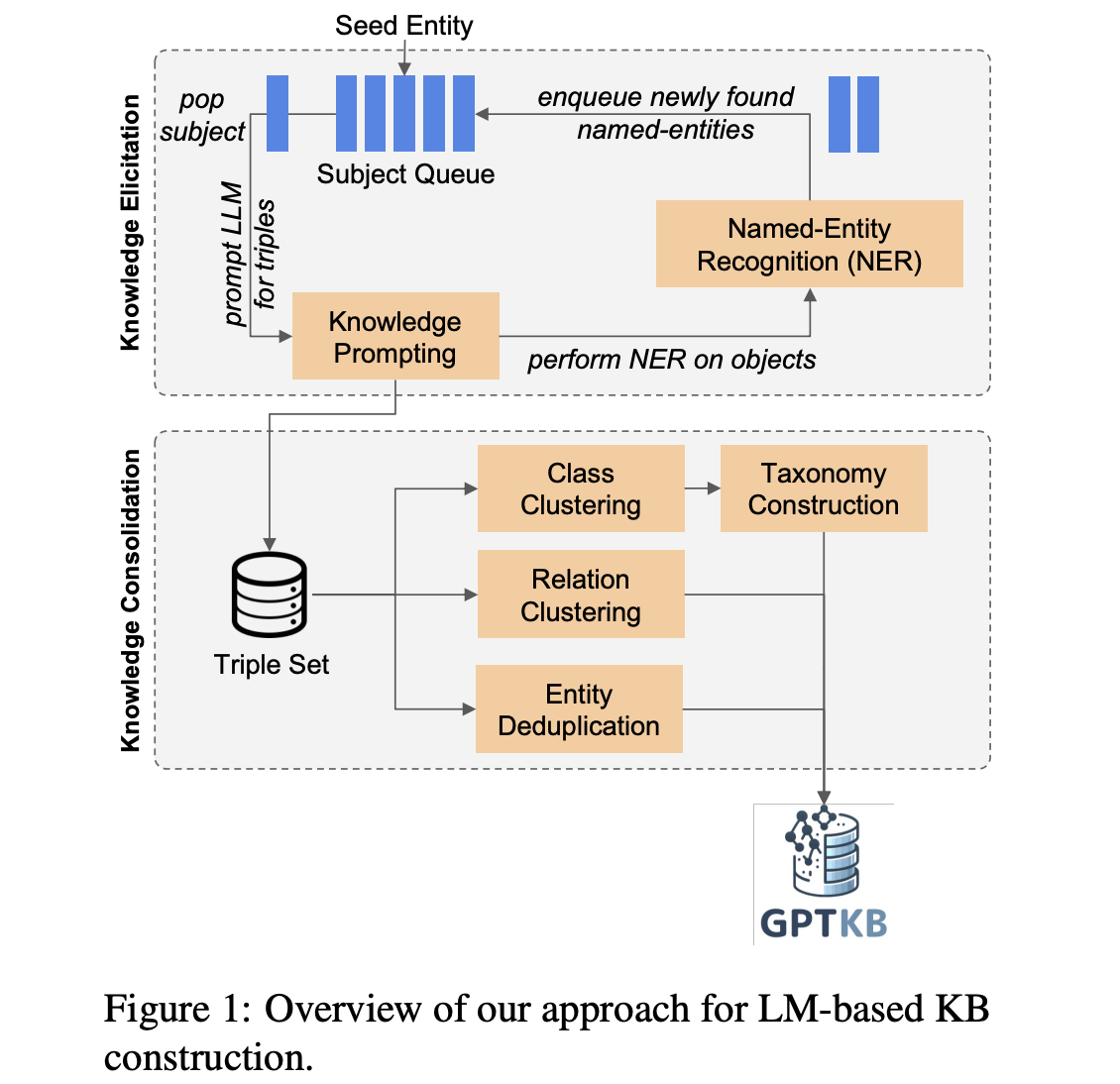
Introduction to Knowledge Base Construction
Knowledge bases like Wikidata, Yago, and DBpedia are essential for intelligent applications. However, the creation of new knowledge bases has slowed down over the last decade. Large Language Models (LLMs) have transformed many AI fields and show promise for providing structured knowledge, but fully extracting and using this knowledge is still a challenge.
Current Challenges
Current methods for building knowledge bases rely on:
- Volunteer-driven models like Wikidata
- Information gathering from sources like Wikipedia, as seen in Yago and DBpedia
- Text-based systems like NELL and ReVerb, which are not widely used
Most evaluations of LLM knowledge are limited, focusing only on specific areas, which fails to capture the full scope of their understanding.
Introducing GPTKB
Researchers from ScaDS.AI, TU Dresden, and the Max Planck Institute have developed GPTKB, a large-scale knowledge base created entirely from LLMs. Built using GPT-4o-mini, GPTKB demonstrates how to extract structured knowledge efficiently, addressing challenges in entity recognition and taxonomy construction.
Key Features of GPTKB
- Contains 105 million triples covering over 2.9 million entities.
- Cost-effective compared to traditional knowledge base construction methods.
- Provides insights into LLM knowledge representation.
How GPTKB Works
GPTKB employs a two-phase approach:
- Phase One: Iterative graph expansion begins with a seed subject and extracts triples while identifying new entities to explore. It uses a multilingual named entity recognition system across 10 languages.
- Phase Two: Focuses on consolidation, including entity canonicalization and relation standardization, operating independently of existing knowledge bases.
Significant Contributions of GPTKB
GPTKB offers diverse knowledge representation, with:
- Nearly 600,000 human entities.
- Properties such as patentCitation and instanceOf.
- New insights, with 69.5% of subjects potentially being novel compared to Wikidata.
Conclusion
The introduction of GPTKB marks a major step forward in knowledge base construction from LLMs. This approach is cost-effective and provides valuable insights into how structured knowledge can be extracted from language models. While there are still challenges, the potential for open-domain knowledge base construction is significant.
Explore Further
Check out the research paper for more details. Follow us for updates on AI solutions and join our community:
Elevate Your Business with AI
Stay competitive and leverage GPTKB for your organization. Here’s how:
- Identify Automation Opportunities: Find key areas where AI can enhance customer interactions.
- Define KPIs: Make sure your AI efforts have measurable impacts.
- Select an AI Solution: Choose tools that fit your needs.
- Implement Gradually: Start small, gather data, and expand wisely.
For AI KPI management advice, connect with us at hello@itinai.com. Stay updated with our insights on AI.