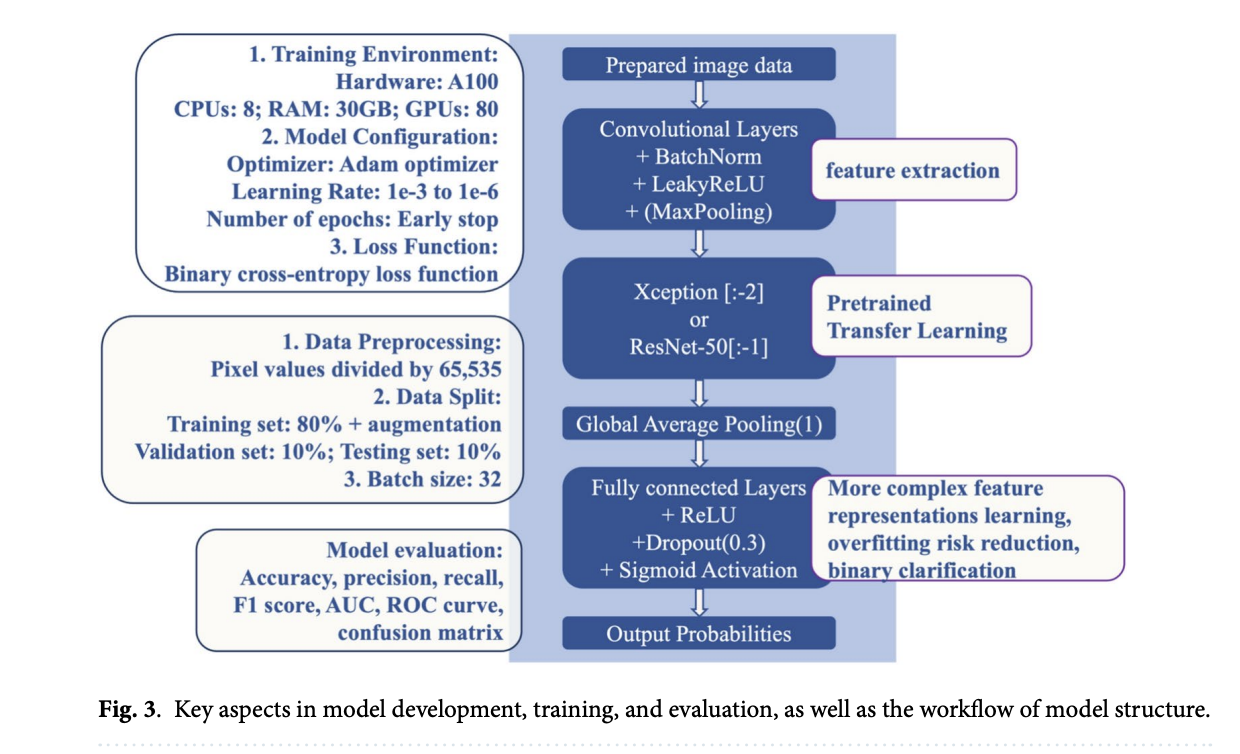
Improving Breast Cancer Diagnosis with AI
Key Challenges in Breast Cancer Diagnosis
Access to mammography datasets and advanced machine-learning techniques is essential for better breast cancer diagnosis. However, researchers face challenges such as:
- Limited access to private datasets
- Selective image sampling from public databases
- Partial code availability
These issues hinder reproducibility and validation, creating barriers for progress in this critical area. In 2022, breast cancer caused 670,000 deaths globally. While technologies like tomosynthesis enhance screening, they still lead to false positives and inconsistencies in radiologists’ interpretations, increasing patient anxiety and healthcare costs.
Innovative Solutions
Researchers from Biomedical Deep Learning LLC and Washington University in St. Louis have created a pilot codebase that simplifies the breast cancer diagnosis process. This solution includes:
- Image preprocessing
- Model development
- Evaluation
The team discovered that using larger input sizes improves malignancy detection accuracy with the CBIS-DDSM mass subset, which offers comprehensive images and regions of interest (ROIs).
Data Processing and Model Training
The CBIS-DDSM dataset features publicly accessible mammography images, expertly curated with updated segmentation and pathology labels. Key features of the codebase include:
- Images converted from DICOM to PNG format
- Focus on abnormal regions with image transformations for enhancement
- A tailored convolutional neural network architecture for model training
Performance metrics such as accuracy, precision, recall, F1 score, and AUROC are used for validation, ensuring optimized results for future research.
Insights from the Study
The research utilized the CBIS-DDSM mass subset, containing 1,696 abnormal ROIs and 1,592 full mammograms. Each image was standardized and augmented to focus on abnormalities. Findings showed:
- Using larger images significantly improved malignant case detection.
- ResNet-50 models outperformed Xception models in accuracy.
- Larger images retained more detail, beneficial for identifying specific cancerous features.
Conclusion and Future Directions
Breast cancer screening has made strides with various innovations; however, challenges remain due to inconsistent methodologies. This study offers a transparent and reproducible codebase to aid model development and validation. By focusing on input size and quality control, researchers aim to enhance model accuracy and reliability, promoting transparency and accelerating advancements in the field.
Get Involved
Explore the full research paper and follow us on social media for updates:
If you’re interested in enhancing your company with AI, consider the following:
- Identify Automation Opportunities
- Define KPIs
- Select an AI Solution
- Implement Gradually
For AI KPI management advice, contact us at hello@itinai.com. Stay tuned for insights on leveraging AI in your operations!