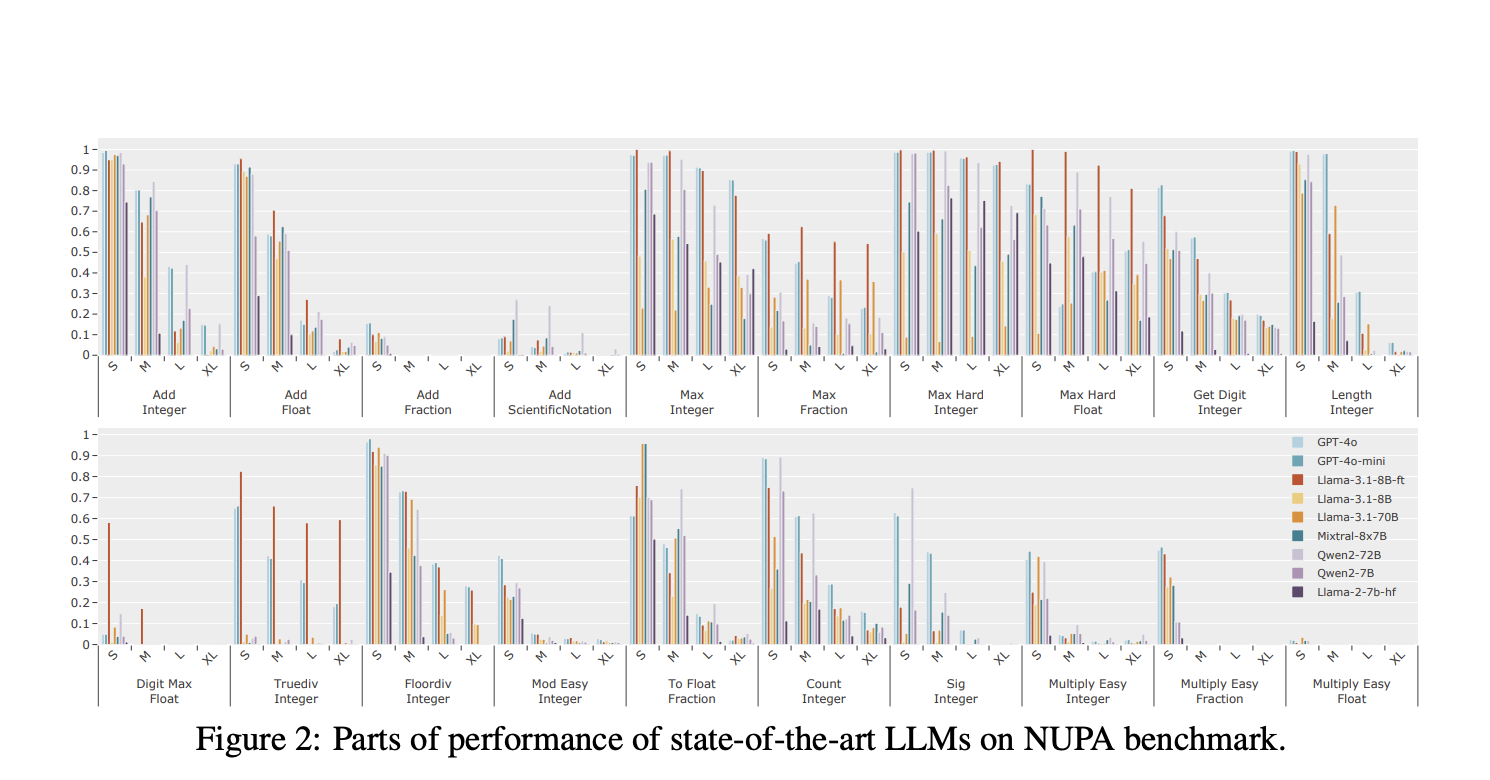
Understanding the Challenges of Large Language Models (LLMs)
Large Language Models (LLMs) have transformed artificial intelligence by excelling in complex reasoning and mathematical tasks. However, they struggle with basic numerical concepts, which are crucial for advanced math skills. Researchers are investigating how LLMs handle numbers like decimals and fractions, highlighting the importance of improving their numerical understanding for fields like finance and physics.
The Core Issue: Numerical Errors
Despite their capabilities, LLMs often make numerical mistakes. For example, they might wrongly compare 9.11 and 9.9 or fail simple arithmetic. These errors undermine their reliability in real-world applications. To address this, we need to enhance the Numerical Understanding and Processing Ability (NUPA) of LLMs, which is vital for arithmetic and broader reasoning.
The Need for Better Evaluation
Current evaluations of LLMs often overlook specific numerical understanding. Tests like GSM8k mix numerical tasks with general reasoning, making it hard to assess LLM performance on numbers alone. By creating targeted benchmarks, researchers can identify weaknesses and improve LLMs for practical numerical tasks that require accuracy and context.
A New Benchmark from Peking University
Researchers at Peking University have developed a specialized benchmark to measure NUPA in LLMs. This benchmark evaluates four numerical formats—integers, fractions, floating-point numbers, and scientific notation—across 17 task categories. It focuses on real-world scenarios and assesses LLMs without relying on external tools.
Pre-Training Techniques for Improvement
The team used various pre-training techniques to evaluate LLM performance and spot weaknesses, such as special tokenizers and positional encoding. Their findings showed that simpler tokenizers provided better accuracy, especially for longer numbers. This research indicates that LLMs need enhancements to process numbers effectively in complex tasks.
Key Findings on Model Performance
The research revealed both strengths and weaknesses in LLMs. For example, models like GPT-4o excelled at simple tasks but struggled with more complex ones, such as scientific notation. Accuracy dropped significantly as task complexity increased, highlighting the need for better numerical processing capabilities.
Addressing Length and Accuracy Challenges
Length also posed challenges, with accuracy decreasing as input length grew. Models often misaligned responses, affecting overall accuracy. The study suggests that improvements in NUPA are necessary to enhance LLM performance in real-world applications.
Conclusion: A Call for Enhanced Methodologies
The findings from Peking University emphasize the need for improved training methods and data to boost numerical reasoning in LLMs. Their work aims to bridge the gap between current capabilities and practical numerical reliability, paving the way for future advancements in AI.
Check out the Paper. All credit for this research goes to the researchers of this project. Also, don’t forget to follow us on Twitter and join our Telegram Channel and LinkedIn Group. If you like our work, you will love our newsletter. Don’t Forget to join our 55k+ ML SubReddit.
Explore AI Solutions for Your Business
If you want to evolve your company with AI and stay competitive, consider the following practical steps:
- Identify Automation Opportunities: Find customer interaction points where AI can add value.
- Define KPIs: Ensure your AI projects have measurable impacts.
- Select an AI Solution: Choose tools that fit your needs and allow for customization.
- Implement Gradually: Start with a pilot project, gather insights, and expand thoughtfully.
For AI KPI management advice, connect with us at hello@itinai.com. For continuous insights into leveraging AI, stay tuned on our Telegram t.me/itinainews or Twitter @itinaicom.
Discover how AI can redefine your sales processes and customer engagement. Explore solutions at itinai.com.