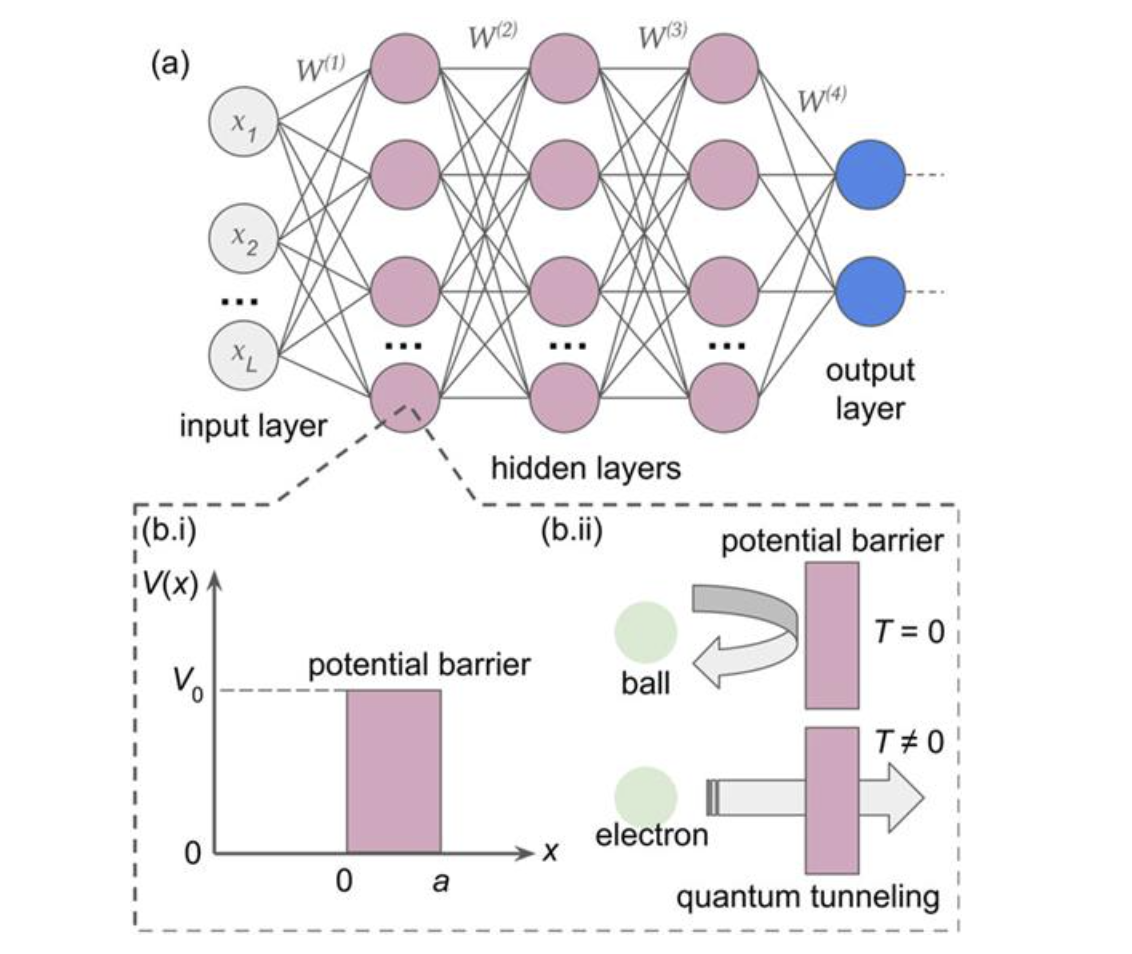
Understanding Quantum Tunneling and AI
The quantum tunneling (QT) effect, discovered in the 1920s, is a key advancement in quantum mechanics. Unlike human brains, artificial intelligence (AI) struggles to interpret complex visual illusions, such as the Necker cube and Rubin’s vase. This challenge arises because AI cannot shift between different interpretations of these illusions like humans do due to fundamental differences in how artificial and biological neurons operate.
Current Limitations in AI Vision
Most AI systems rely on traditional deep neural networks (DNNs) with standard activation functions (like ReLU) to process visual information. However, DNNs often fall short of mimicking human vision, which is influenced by physical, physiological, and psychological factors. This makes it difficult for them to respond like humans, who can easily switch perceptions in response to a visual stimulus. Unlike human brains, which exhibit quantum-like behavior, traditional DNNs do not have this capability.
Introducing QT-DNN: A New Approach
Researchers at Charles Sturt University, Australia, have developed a revolutionary neuromorphic DNN model called QT-DNN, which utilizes quantum tunneling as its activation function. This model has been tested specifically on the Necker Cube and Rubin’s vase illusions. The key innovation involves replacing conventional activation functions with quantum tunneling probability equations, allowing the model to process visual information without bias, thanks to a unique quantum random number generator.
QT-DNN Architecture and Benefits
The QT-DNN model features an architecture with:
- 100 input nodes
- Three hidden layers with 20 nodes each
- Two output nodes for classification
This setup not only allows for switching between different interpretations of illusions but also facilitates the representation of intermediate states, showcasing multiple perceptions—which is a natural ability for humans but challenging for classic DNNs. QT-DNN demonstrates improved alignment with theoretical quantum models and human perception observations compared to traditional DNNs.
Practical Applications and Future Potential
QT-DNN, based on quantum mechanics, presents a groundbreaking way to bridge the gap between machine and human perception. Its applications are particularly valuable in sectors that require human-like visual processing, including:
- Aviation safety
- Augmented reality systems
- Medical diagnostics
This research paves the way for creating more advanced AI systems capable of better interpreting visual information akin to human perception.
Stay Connected and Explore More
For more insights, check out the research paper and details. Follow us on Twitter, join our Telegram Channel, and participate in our LinkedIn Group. If you appreciate our work, subscribe to our newsletter. Join over 55k members in our ML SubReddit!
Sponsorship Opportunities
If you’re interested in promoting your research, product, or webinar to our audience of over 1 million monthly readers and 500k community members, we invite you to explore our sponsorship options.
To enhance your company’s AI capabilities and remain competitive, take action with QT-DNN and discover how AI can transform your workflow. Identify automation opportunities, define measurable KPIs, select the right AI tools, and implement them gradually.
For advice on AI KPI management, connect with us at hello@itinai.com. Stay informed by following us on Telegram t.me/itinainews or Twitter @itinaicom.
Discover how AI can revolutionize your sales processes and customer engagement by exploring our solutions at itinai.com.