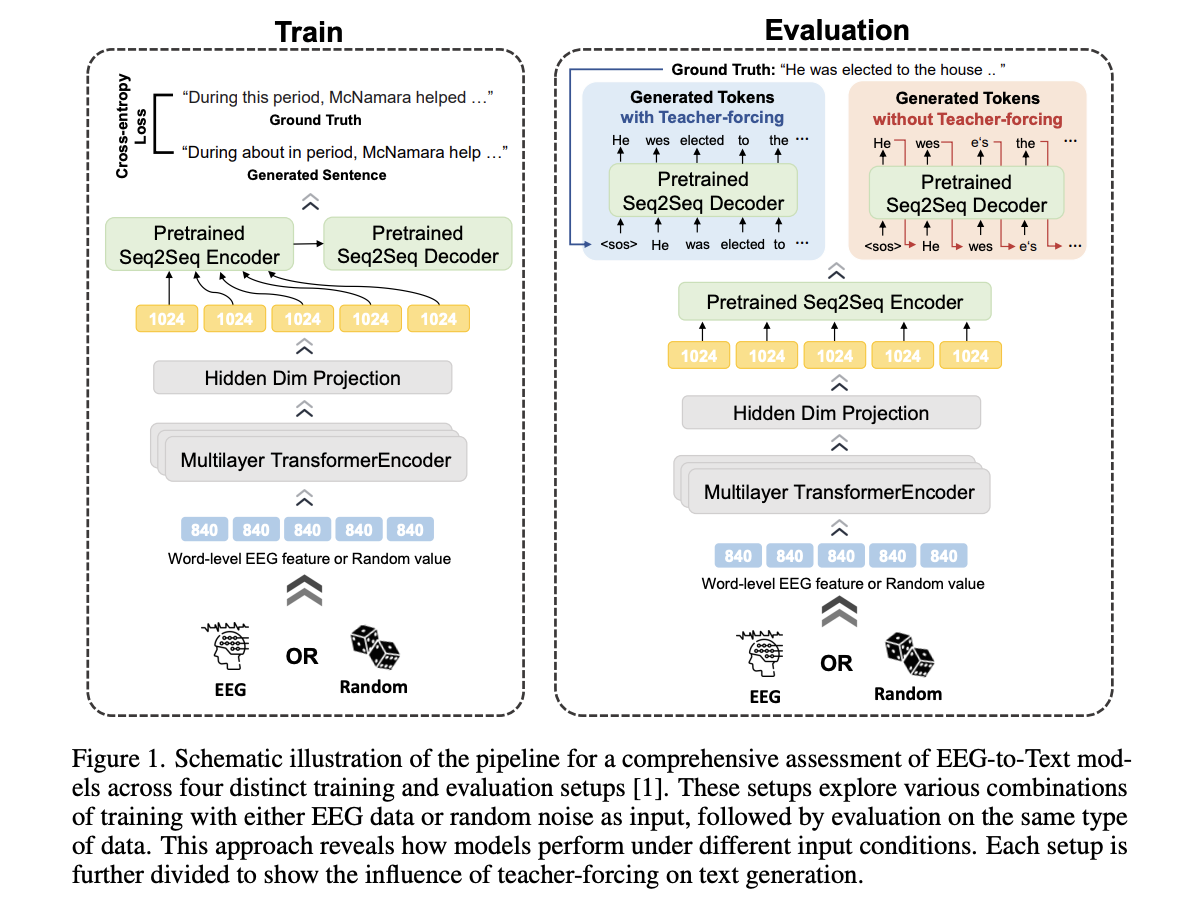
Understanding EEG-to-Text Models
The Challenge
One major issue with EEG-to-Text models is ensuring they truly learn from EEG signals instead of just memorizing text patterns. Many studies report impressive results, but they often use methods that can misrepresent the model’s actual performance. This can lead to inflated success rates, masking the model’s real learning capabilities. Additionally, current research lacks a crucial test: evaluating how models perform with random noise. This is vital to differentiate between models that genuinely decode EEG signals and those that simply recall memorized data. Addressing this challenge is essential for creating reliable EEG-to-Text systems, especially for individuals with disabilities who depend on these technologies for communication.
Current Approaches
Most existing methods utilize encoder-decoder architectures with pre-trained models like BART, PEGASUS, and T5. These models use advanced techniques to convert EEG signals into text, evaluated through metrics like BLEU and ROUGE. However, the reliance on teacher forcing can distort performance scores, making it unclear whether the models are genuinely learning from EEG data or just reproducing memorized sequences. This limitation affects the reliability of these models in real-world applications, highlighting the need for better evaluation methods that accurately reflect their learning effectiveness.
A New Assessment Framework
Researchers from Kyung Hee University and the Australian Artificial Intelligence Institute have developed a more robust evaluation framework to tackle these issues. Their approach includes four experimental scenarios:
- Training and testing on EEG data
- Training and testing with random noise only
- Training with EEG but testing on noise
- Training on noise but testing on EEG data
By comparing performance across these scenarios, researchers can determine whether models are learning meaningful information from EEG signals or simply memorizing patterns. This methodology also tests various pre-trained transformer models to assess how different architectures impact performance, leading to more reliable EEG-to-Text evaluations.
Experimental Insights
The experiments utilized two datasets, ZuCo 1.0 and ZuCo 2.0, which contain EEG data collected during natural reading activities. EEG signals were processed to extract 840 features per word based on eye movements, using eight specific frequency bands for comprehensive analysis. The data was split into 80% for training, 10% for development, and 10% for testing, with training conducted over 30 epochs on advanced GPUs. Performance metrics included BLEU, ROUGE, and WER, providing a solid framework to assess the proposed method’s effectiveness in real learning conditions.
Key Findings
The evaluation showed that models scored significantly higher with teacher forcing, sometimes inflating perceived performance by up to three times. For example, without teacher forcing, the BLEU-1 score for EEG-trained models dropped drastically, suggesting that these models may not truly understand the input. Surprisingly, performance was similar whether the input was EEG data or random noise, indicating a reliance on memorized patterns rather than genuine learning. This underscores the urgent need for evaluation techniques that avoid teacher forcing and incorporate noise baselines to accurately measure learning from EEG data.
Conclusion
This research sets new standards for evaluating EEG-to-Text models through rigorous benchmarking practices, ensuring that real learning occurs from EEG inputs. By introducing diverse training and testing scenarios, the authors address long-standing issues related to teacher forcing and memorization, allowing for a clearer distinction between actual learning and memorized patterns. This work lays the groundwork for developing more effective EEG-to-Text models, which can significantly enhance communication systems for individuals with impairments. Emphasizing transparent reporting and strict baselines will foster trust in EEG-to-Text research, paving the way for advancements that can reliably harness the true potential of these models for effective communication solutions.
Stay Connected
Check out the Paper. All credit for this research goes to the researchers of this project. Also, don’t forget to follow us on Twitter and join our Telegram Channel and LinkedIn Group. If you like our work, you will love our newsletter. Don’t Forget to join our 55k+ ML SubReddit.
Explore AI Solutions
If you want to evolve your company with AI, stay competitive, and leverage its advantages, discover how AI can transform your work processes. Here are some practical steps:
- Identify Automation Opportunities: Find key customer interaction points that can benefit from AI.
- Define KPIs: Ensure your AI initiatives have measurable impacts on business outcomes.
- Select an AI Solution: Choose tools that align with your needs and allow for customization.
- Implement Gradually: Start with a pilot project, gather data, and expand AI usage wisely.
For AI KPI management advice, connect with us at hello@itinai.com. For continuous insights into leveraging AI, stay tuned on our Telegram channel or Twitter.
Discover More
Explore how AI can redefine your sales processes and customer engagement. Visit itinai.com for more solutions.