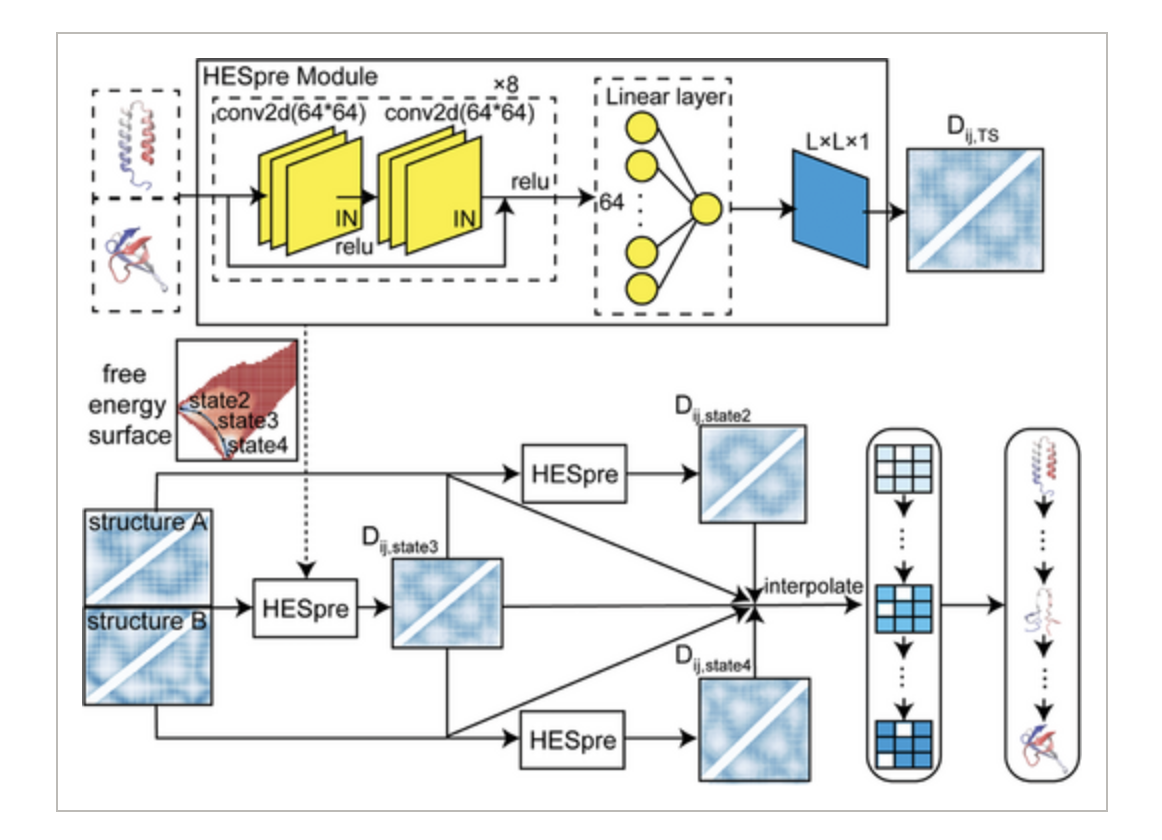
Understanding Protein Conformational Changes
Predicting how proteins change shape is a major challenge in computational biology and artificial intelligence. While deep learning advancements like AlphaFold2 have improved predictions of static protein structures, they do not effectively address the dynamic changes proteins undergo to perform their biological functions. These changes are essential for understanding various biological processes, such as enzyme activity and signal transduction.
Challenges in Current Models
Current models for predicting protein transitions, like elastic network analysis and hybrid models, work well for simple movements but struggle with the complex changes seen in larger proteins. Recent deep learning methods, including auto-encoders and diffusion models, have been developed but often rely on linear pathways, which do not apply to complex transitions. Additionally, these models require a lot of data and are computationally expensive, making them less practical for real-time applications.
A Novel Solution: PATHpre
The authors introduce a new deep learning approach called PATHpre, which uses high-throughput biophysical sampling to overcome the lack of data on protein conformational transitions. By combining molecular dynamics simulations with enhanced sampling, they created a dataset of 2,635 proteins with two known states. PATHpre predicts structural pathways for conformational changes with high accuracy, without relying on linear assumptions.
How PATHpre Works
PATHpre uses distance matrices from two conformational states and applies convolutional neural networks to identify high-energy states between these states. It focuses on unique contacts that occur during transitions, providing insights into inter-domain and intra-domain movements, localized unfolding, and global fold changes. The model has been validated across various proteins, showing strong correlations and low errors, making it versatile for different structural classes.
Performance and Applicability
PATHpre has demonstrated high accuracy in predicting protein transition pathways, aligning well with experimental and simulated data. It effectively captures both simple and complex conformational changes, proving reliable across different protein lengths and structures. Notably, it outperformed traditional hybrid approaches in challenging scenarios, confirming its broad applicability.
Significance of the Research
This research marks a significant advancement in AI-driven protein modeling, offering a scalable and data-efficient method for predicting protein conformational transitions. By integrating large-scale biophysical sampling with deep learning, PATHpre addresses the critical challenge of limited data and captures nonlinear transitions across diverse proteins. This model will enhance AI applications in computational biology, serving as a powerful tool for exploring dynamic protein behavior in various contexts, from drug discovery to synthetic biology.
Get Involved
Check out the Paper. All credit for this research goes to the researchers involved. Follow us on Twitter, join our Telegram Channel, and connect with our LinkedIn Group. If you appreciate our work, subscribe to our newsletter and join our 55k+ ML SubReddit.
Sponsorship Opportunity
Promote your research, product, or webinar to over 1 million monthly readers and 500k+ community members.
Transform Your Business with AI
Stay competitive and leverage AI to evolve your company. Here’s how:
- Identify Automation Opportunities: Find key customer interaction points that can benefit from AI.
- Define KPIs: Ensure your AI initiatives have measurable impacts on business outcomes.
- Select an AI Solution: Choose tools that fit your needs and allow for customization.
- Implement Gradually: Start with a pilot project, gather data, and expand AI usage carefully.
For AI KPI management advice, connect with us at hello@itinai.com. For ongoing insights into leveraging AI, follow us on Telegram or @itinaicom.
Discover how AI can transform your sales processes and customer engagement. Explore solutions at itinai.com.