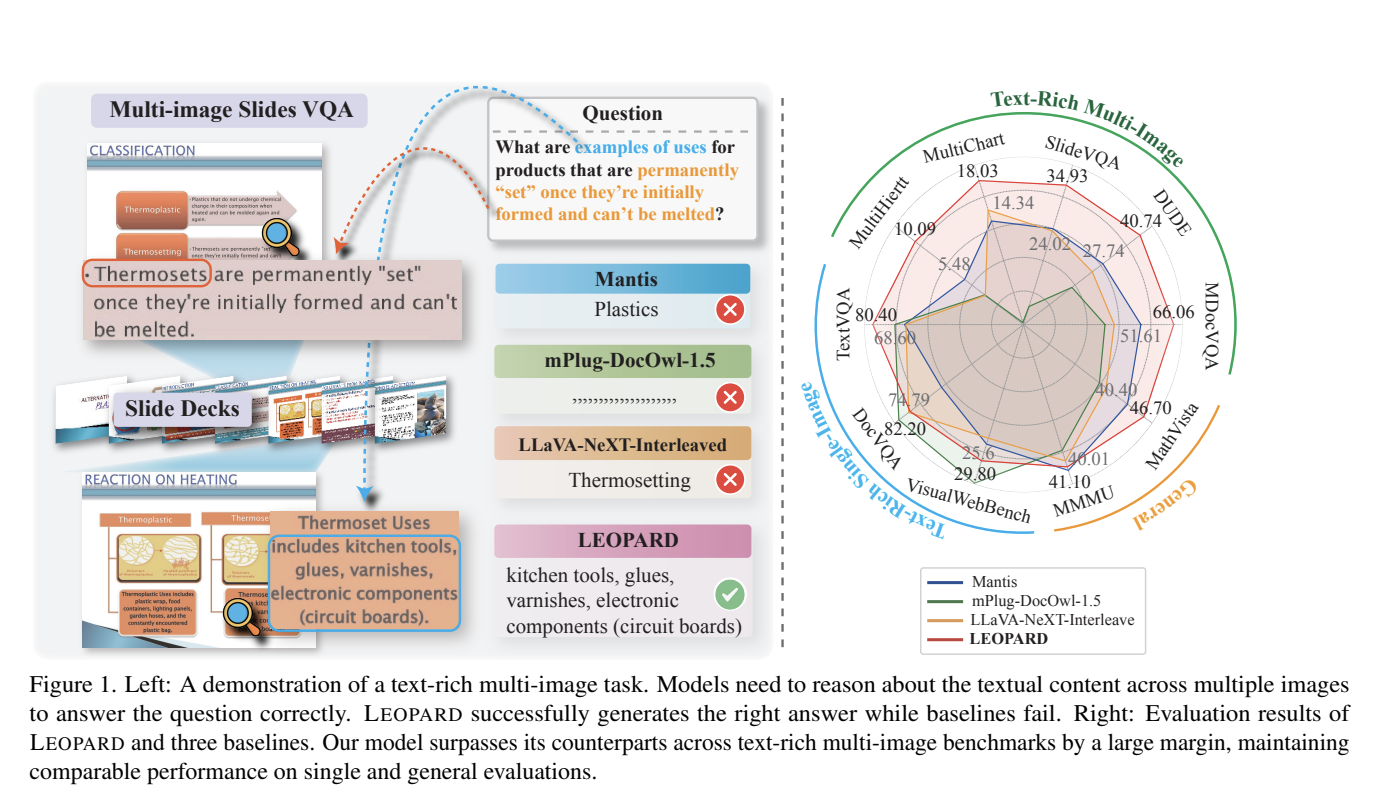
Introduction to Leopard: A New AI Solution
In recent years, multimodal large language models (MLLMs) have transformed how we handle tasks that combine vision and language, such as image captioning and object detection. However, existing models struggle with text-rich images, which are essential for applications like presentation slides and scanned documents. This is where Leopard comes in.
Challenges in Current MLLMs
Current MLLMs, like LLaVAR and mPlug-DocOwl-1.5, face two main challenges:
- Lack of Quality Datasets: There are not enough high-quality instruction-tuning datasets for multi-image scenarios.
- Image Resolution Issues: Maintaining the right balance between image resolution and visual sequence length is difficult.
Addressing these challenges is crucial for real-world applications that rely on understanding text-rich content.
Leopard: A Tailored Solution
Researchers from the University of Notre Dame, Tencent AI Seattle Lab, and the University of Illinois Urbana-Champaign have developed Leopard, an MLLM specifically designed for vision-language tasks involving multiple text-rich images. Here’s what makes Leopard unique:
- Extensive Dataset: Leopard uses a dataset of about one million high-quality multimodal instruction-tuning data points, focusing on multi-page documents, tables, and web snapshots.
- Adaptive Encoding Module: This feature optimizes image resolution and sequence length dynamically, ensuring high-quality detail is preserved.
Key Advantages of Leopard
Leopard stands out due to its innovative features:
- High-Resolution Detail: The adaptive encoding module maintains image quality while efficiently managing sequence lengths, preventing information loss.
- Pixel Shuffling: This technique compresses long visual sequences into shorter, lossless ones, enhancing the model’s ability to handle complex visual inputs.
Real-World Applications
Leopard significantly outperforms previous models like OpenFlamingo and VILA in practical scenarios. Benchmark tests show an average improvement of over 9.61 points on key tasks involving multiple text-rich images, such as SlideVQA and Multi-page DocVQA. This capability is invaluable for:
- Understanding multi-page documents
- Analyzing presentations in business and education
Conclusion
Leopard marks a significant advancement in multimodal AI, particularly for tasks involving multiple text-rich images. By overcoming the challenges of limited datasets and balancing image resolution, Leopard offers a powerful solution for processing complex visual information. Its superior performance and innovative encoding approach highlight its potential impact across various real-world applications.
Get Involved
Check out the Leopard Paper and Leopard Instruct Dataset on HuggingFace. Follow us on Twitter, join our Telegram Channel, and connect with our LinkedIn Group. If you enjoy our work, subscribe to our newsletter and join our 55k+ ML SubReddit.
Explore AI Solutions for Your Business
To stay competitive, consider using Leopard for your AI needs. Here’s how to get started:
- Identify Automation Opportunities: Find key customer interaction points that can benefit from AI.
- Define KPIs: Ensure your AI efforts have measurable impacts on business outcomes.
- Select an AI Solution: Choose tools that fit your needs and allow for customization.
- Implement Gradually: Start with a pilot project, gather data, and expand AI usage wisely.
For AI KPI management advice, contact us at hello@itinai.com. For ongoing insights into leveraging AI, follow us on Telegram or Twitter.