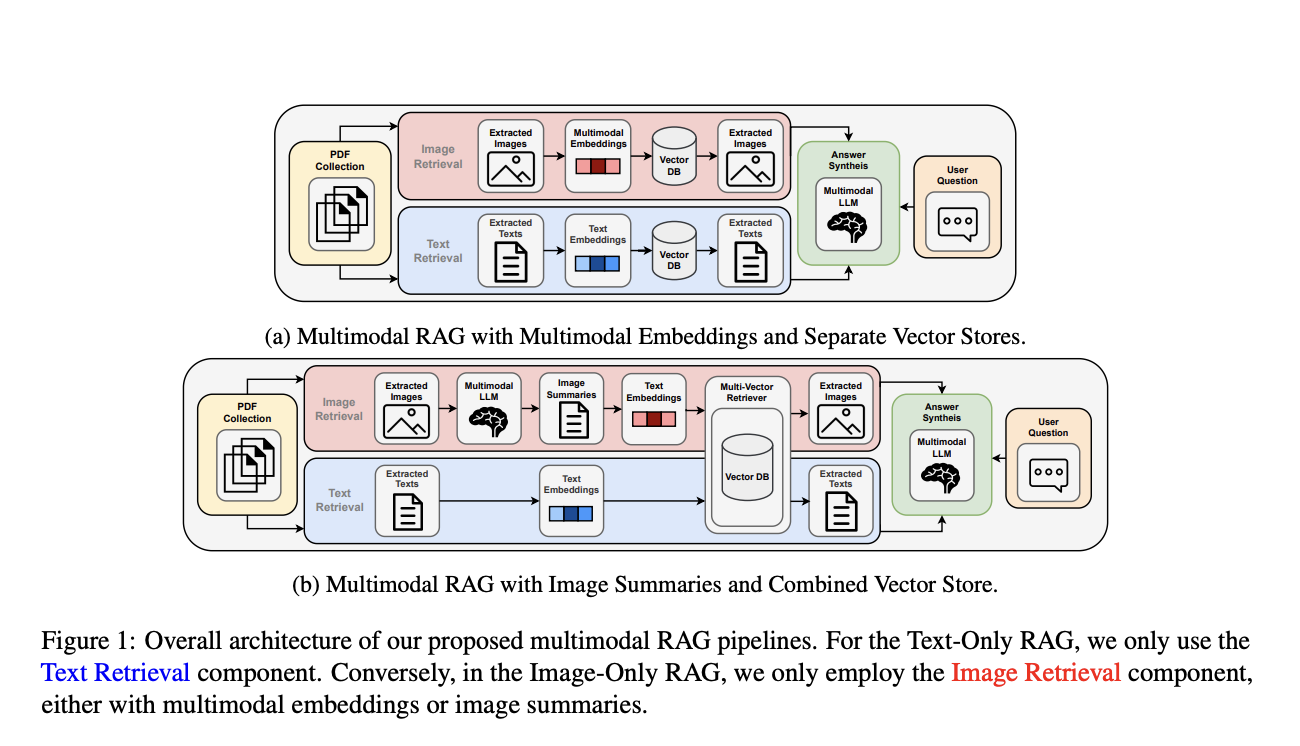
Unlocking AI Potential in Industry with Multimodal RAG Technology
What is Multimodal RAG?
Multimodal Retrieval Augmented Generation (RAG) technology enhances AI applications in manufacturing, engineering, and maintenance. It effectively combines text and images from complex documents like manuals and diagrams, improving task accuracy and efficiency.
Challenges in Industrial AI
AI systems often struggle to provide accurate answers when interpreting both text and visuals. Traditional models may lack the specific knowledge needed for industrial tasks, leading to inaccuracies. This highlights the need for solutions that integrate text and image data effectively.
Current Limitations
Most existing systems focus on either text or images separately, creating gaps in handling documents that require both. Text-only models may miss critical visual elements, while image-only approaches often fall short in industrial contexts.
Innovative Solutions from LMU Munich and Siemens
Researchers have developed a multimodal RAG system using advanced models like GPT-4 Vision and LLaVA. This system employs two strategies for image data:
– **Multimodal embeddings**: Aligns text and images in a shared space for better retrieval.
– **Image-based textual summaries**: Converts visuals into descriptive text, ensuring comprehensive information access.
How the System Works
The multimodal RAG system retrieves and interprets data more accurately by:
– Embedding text from documents for relevant response generation.
– Using CLIP to match images with textual queries, enhancing cross-modal understanding.
– Processing images into concise summaries for easier retrieval while retaining original visuals.
Performance Improvements
The multimodal RAG system shows significant improvements in handling complex queries. Accuracy increased by nearly 80% when images were included. The image-summary method outperformed other techniques in providing relevant context.
Future of Multimodal RAG in Industry
This research demonstrates that integrating multimodal RAG can greatly enhance AI performance in industries requiring both visual and textual interpretation. It opens up exciting possibilities for future advancements in AI applications.
Stay Connected
For more insights, check out the research paper and follow us on Twitter, join our Telegram Channel, and LinkedIn Group. If you appreciate our work, subscribe to our newsletter and join our 55k+ ML SubReddit community.
Transform Your Business with AI
To stay competitive, consider these steps:
– **Identify Automation Opportunities**: Find areas in customer interactions that can benefit from AI.
– **Define KPIs**: Ensure measurable impacts from your AI initiatives.
– **Select an AI Solution**: Choose tools that fit your needs and allow customization.
– **Implement Gradually**: Start small, gather data, and expand wisely.
For AI KPI management advice, connect with us at hello@itinai.com. For continuous insights, follow us on Telegram or Twitter. Discover how AI can transform your sales processes and customer engagement at itinai.com.