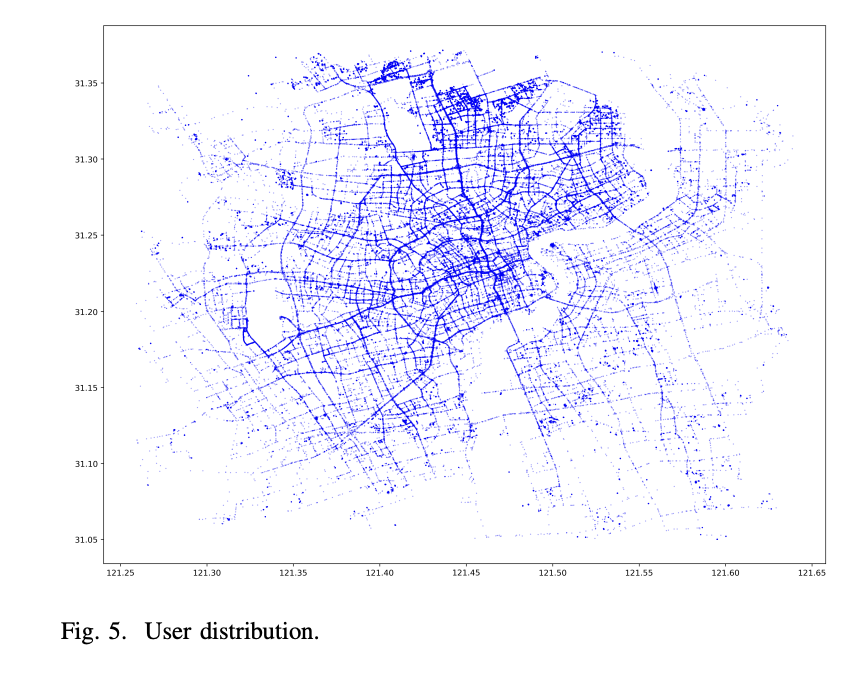
Understanding Quality of Service (QoS)
Quality of Service (QoS) is crucial for assessing how well network services perform, especially in mobile environments where devices frequently connect to edge servers. Key aspects of QoS include:
- Bandwidth
- Latency
- Jitter
- Data Packet Loss Rate
The Challenge with Current QoS Datasets
Most existing QoS datasets, like the WS-Dream dataset, focus on fixed metrics and often ignore important factors such as:
- Geographic Location
- Time Variability
These dynamic factors are essential for accurately predicting network performance, as QoS can change based on location and time.
Limitations of Current Prediction Methods
Current QoS prediction techniques mainly use collaborative filtering, which relies on past user data. However, they struggle with:
- Data Sparsity – This limits accurate predictions.
- Ignoring Temporal and Spatial Variations
Deep learning methods have been introduced but still need adjustments to adapt to the dynamic nature of mobile environments.
Introducing CHESTNUT Dataset
The CHESTNUT dataset was developed by researchers from Shanghai University to enhance QoS prediction. It includes critical factors such as:
- Edge Server Load
- User Mobility
- Service Diversity
These elements are vital for modeling interactions in mobile edge environments accurately.
How CHESTNUT Works
CHESTNUT utilizes two real-world datasets:
- Johnson Taxi GPS Dataset – Simulates user mobility.
- Shanghai Telecom Dataset – Represents edge server locations.
After processing these datasets, CHESTNUT provides a realistic view of user and server behaviors, including:
- Response Time
- Network Jitter
This dataset allows for more precise QoS predictions by incorporating real-world dynamics and resource-based attributes.
Conclusion
The CHESTNUT dataset significantly improves QoS prediction for mobile edge environments by integrating dynamic temporal and geographic information. This comprehensive approach aims to create more accurate and efficient QoS models, filling the gaps left by traditional datasets.
It has been found that response time is affected by the load and resource demands of edge servers, which is critical for future QoS predictions.
Stay Connected
Check out the Paper for more details. Follow us on Twitter, join our Telegram Channel, and connect with our LinkedIn Group. If you appreciate our work, subscribe to our newsletter. Also, join our 55k+ ML SubReddit.
Leverage AI for Your Business
To stay competitive, consider using the CHESTNUT dataset for your AI solutions:
- Identify Automation Opportunities – Find key customer interactions that can benefit from AI.
- Define KPIs – Ensure measurable impacts on business outcomes.
- Select an AI Solution – Choose tools that fit your needs.
- Implement Gradually – Start small, gather data, and expand wisely.
For AI KPI management advice, contact us at hello@itinai.com. For continuous insights, follow us on Telegram or Twitter.
Discover how AI can transform your sales processes and customer engagement at itinai.com.