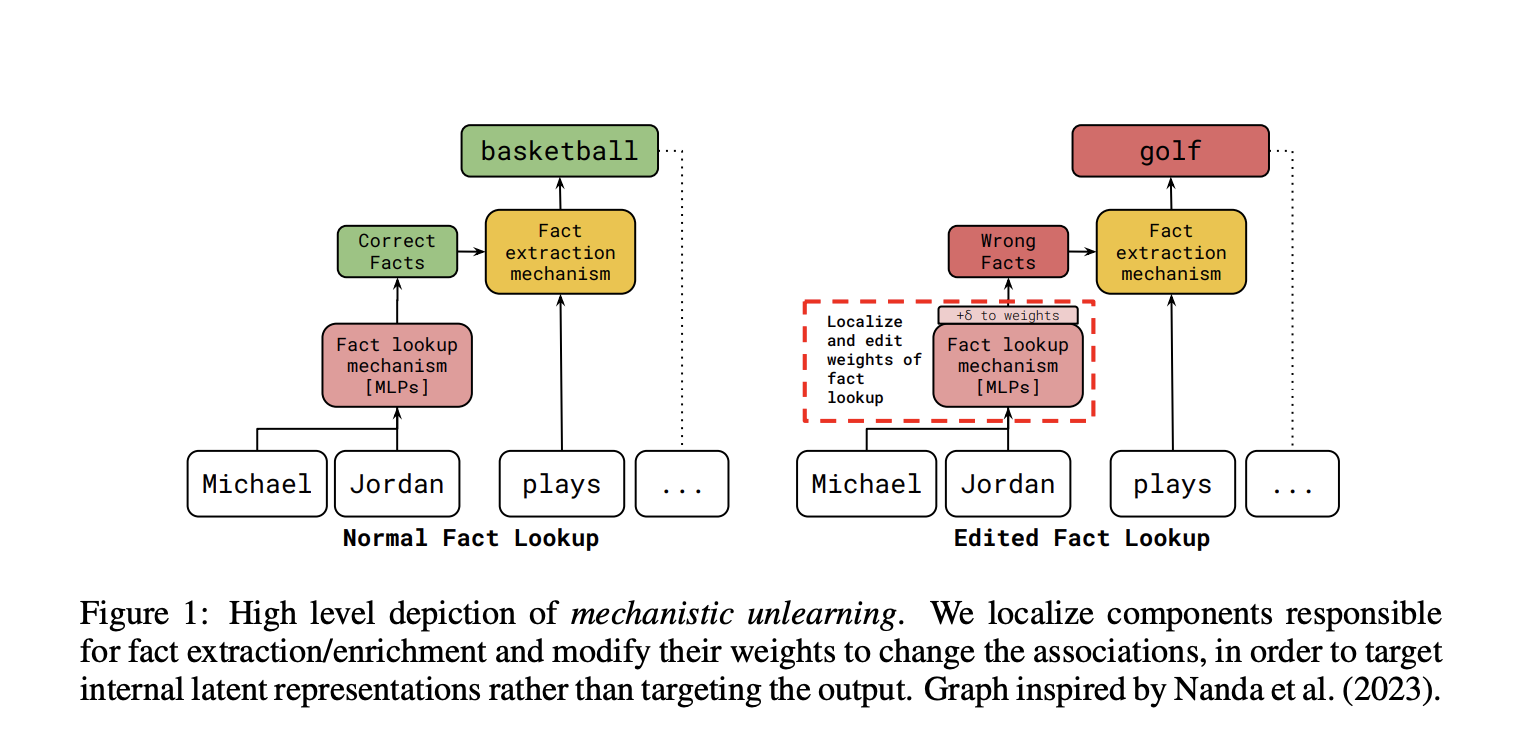
Understanding Mechanistic Unlearning in AI
Challenges with Large Language Models (LLMs)
Large language models can sometimes learn unwanted information, making it crucial to adjust or remove this knowledge to maintain accuracy and control. However, editing or “unlearning” specific knowledge is challenging. Traditional methods can unintentionally affect other important information, leading to a loss of overall model performance.
Current Solutions and Their Limitations
Researchers are exploring methods like causal tracing and attribution patching to identify and edit crucial components in AI models. While these methods aim to enhance safety and fairness, they often struggle with robustness. Changes may not be permanent, and models can revert to unwanted knowledge, sometimes producing harmful responses.
Introducing Mechanistic Unlearning
A team from the University of Maryland, Georgia Institute of Technology, University of Bristol, and Google DeepMind has proposed a new method called Mechanistic Unlearning. This approach uses mechanistic interpretability to accurately locate and edit specific components related to factual recall, leading to more reliable and effective edits.
Research Findings
The study tested unlearning methods on two datasets: Sports Facts and CounterFact. They successfully altered associations with athletes and swapped correct answers for incorrect ones. By focusing on specific model parts, they achieved better results with fewer changes, ensuring unwanted knowledge is effectively removed and less likely to return.
Benefits of Mechanistic Unlearning
- Robust Edits: The method provides stronger and more reliable knowledge unlearning.
- Reduced Side Effects: It minimizes unintended impacts on other model capabilities.
- Improved Accuracy: Manual localization techniques enhance performance in tasks like multiple-choice tests.
Conclusion
This research presents a promising solution for robust knowledge unlearning in LLMs. By precisely targeting model components, Mechanistic Unlearning enhances the effectiveness of the unlearning process and opens up new avenues for interpretability methods.
Stay Connected
Check out the full paper for more details. Follow us on Twitter, join our Telegram Channel, and connect with our LinkedIn Group for updates. If you enjoy our insights, subscribe to our newsletter and join our 55k+ ML SubReddit community.
Upcoming Webinar
Join us on Oct 29, 2024: Discover the best platform for serving fine-tuned models with the Predibase Inference Engine.
Transform Your Business with AI
Leverage Mechanistic Unlearning to stay competitive and redefine your operations:
- Identify Automation Opportunities: Find customer interaction points that can benefit from AI.
- Define KPIs: Ensure measurable impacts from your AI initiatives.
- Select an AI Solution: Choose tools that fit your needs and allow customization.
- Implement Gradually: Start small, gather data, and expand wisely.
For AI KPI management advice, contact us at hello@itinai.com. For ongoing insights, follow us on Telegram or Twitter @itinaicom.
Discover how AI can enhance your sales processes and customer engagement at itinai.com.