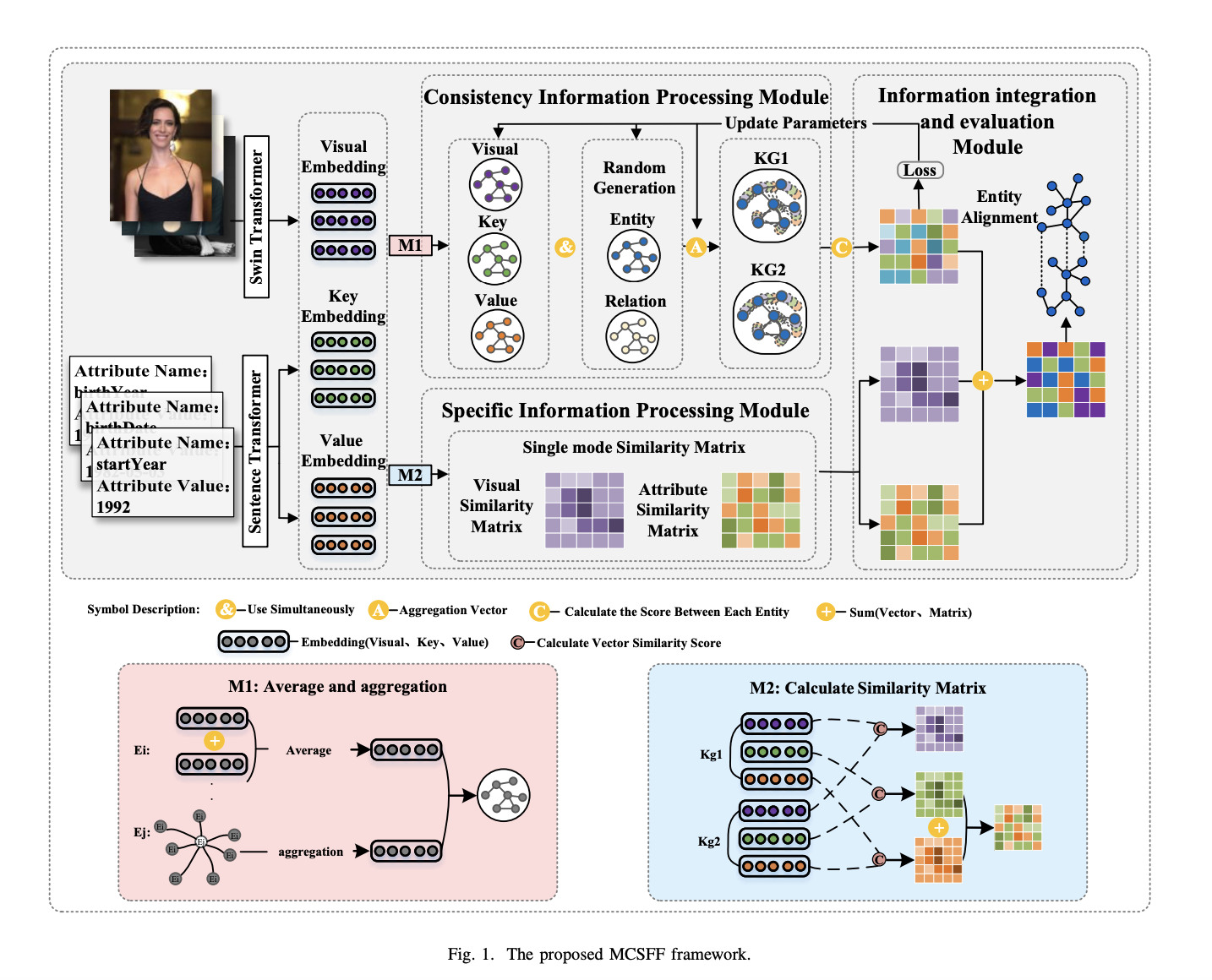
Understanding Multi-modal Entity Alignment (MMEA)
Multi-modal entity alignment (MMEA) is a method that uses information from different sources to match related entities across various knowledge graphs. By integrating data from text, structure, attributes, and external sources, MMEA improves accuracy and effectiveness compared to single-source methods. However, it faces challenges like data sparsity, noise, and the complexity of combining different types of information.
Current Challenges in MMEA
Current MMEA techniques, such as MtransE and GCN-Align, often focus too much on shared features between data types and overlook their unique aspects. This can lead to a loss of important information and lower accuracy in matching entities. The main challenge is effectively merging visual and attribute knowledge while keeping each data type’s specific characteristics intact.
Introducing the MCSFF Framework
Researchers from Central South University of Forestry and Technology in China have developed a new solution: the Multi-modal Consistency and Specificity Fusion Framework (MCSFF). This framework improves entity alignment by capturing consistent information across different data types while maintaining their unique features. It uses advanced infrastructure to optimize resource use in processing large datasets.
How MCSFF Works
The MCSFF framework consists of three main components:
- Single-modality similarity matrix computation: This module calculates the similarity between entities based on visual and attribute data, preserving each type’s unique traits.
- Cross-modal consistency integration (CMCI): This method enhances the quality of features by combining information across data types, resulting in more accurate entity representations.
- Iterative embedding update process: This step refines the entity features by aggregating information from related entities using an attention mechanism.
Performance and Benefits of MCSFF
The MCSFF framework significantly outperforms existing methods in multi-modal entity alignment tasks, showing improvements in key metrics like Hits@1, Hits@10, and MRR. For example, it surpassed the best existing method by up to 4.9% in Hits@10. Studies indicate that essential components like CMCI and the Single-Modality Similarity Matrix are crucial for its success.
Conclusion
MCSFF effectively addresses the shortcomings of current MMEA methods by capturing both consistency and specificity across data types. This leads to improved accuracy and robustness, especially in situations with limited training data. Its strong performance makes it a valuable tool for large-scale entity alignment tasks.
Get Involved
Check out the Paper for more details. Follow us on Twitter, join our Telegram Channel, and connect with our LinkedIn Group. If you appreciate our work, subscribe to our newsletter and join our 55k+ ML SubReddit.
Upcoming Live Webinar
Upcoming Live Webinar- Oct 29, 2024: The Best Platform for Serving Fine-Tuned Models: Predibase Inference Engine.
Transform Your Business with AI
To stay competitive, consider implementing the MCSFF Framework. Here are practical steps to harness AI:
- Identify Automation Opportunities: Find customer interaction points that can benefit from AI.
- Define KPIs: Ensure your AI projects have measurable impacts.
- Select an AI Solution: Choose tools that fit your needs and allow customization.
- Implement Gradually: Start with a pilot project, gather insights, and expand wisely.
For AI KPI management advice, contact us at hello@itinai.com. Stay updated on AI insights via our Telegram at t.me/itinainews or Twitter @itinaicom.
Enhance Your Sales and Customer Engagement with AI
Discover innovative solutions at itinai.com.