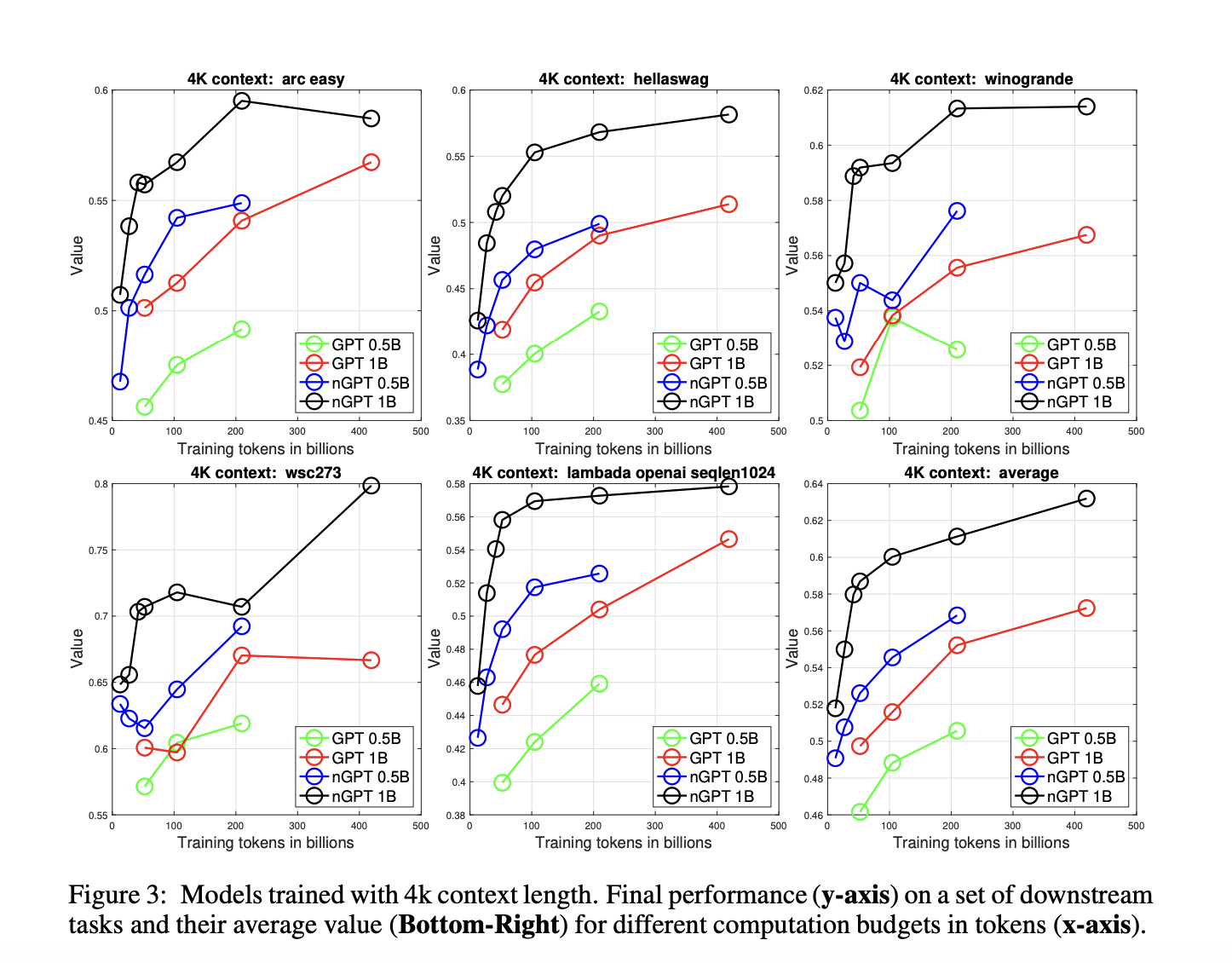
The Normalized Transformer (nGPT) – A New Era in AI Training
Understanding the Challenge
The rise of Transformer models has greatly improved natural language processing. However, training these models can be slow and resource-heavy. This research aims to make training more efficient while keeping performance high. It focuses on integrating normalization into the Transformer architecture for better results.
Introducing the Normalized Transformer (nGPT)
NVIDIA researchers have developed the Normalized Transformer, or nGPT. This model uses a unique method of representation learning on a hypersphere. By normalizing all vectors in the model, like embeddings and hidden states, nGPT allows data to move effectively across the hypersphere’s surface. This leads to faster and more stable training. nGPT can reduce the training steps by 4 to 20 times, depending on sequence length.
Key Features of nGPT
– **Systematic Normalization:** All components are constrained to a hypersphere, ensuring a consistent representation.
– **Cosine Similarity:** Vector operations are treated as dot products, enhancing the model’s ability to learn.
– **Learnable Scaling Parameters:** Instead of traditional methods, nGPT uses adjustable parameters to control normalization.
– **Adaptive Learning Rates:** The training process is optimized with learning rates that adapt to each layer.
Impressive Results
Experiments using the OpenWebText dataset show that nGPT outperforms standard GPT models. For example, with a context length of 4k tokens, nGPT achieved the same validation loss as GPT with only one-tenth of the iterations. It consistently excels in various downstream tasks, providing quicker training and better accuracy.
Conclusion and Future Potential
The Normalized Transformer represents a significant leap in training large language models efficiently. By combining previous findings on normalization and embedding, nGPT offers a more resource-effective solution without sacrificing performance. This approach could lead to enhancements in complex models and hybrid frameworks.
Stay Connected and Learn More
Check out the research paper for in-depth information. Follow us on Twitter, join our Telegram Channel, and connect with our LinkedIn Group. If you enjoy our insights, subscribe to our newsletter and join our 50k+ ML SubReddit.
Upcoming Live Webinar
Join us on Oct 29, 2024, to learn how to enhance inference throughput by 4x while cutting serving costs by 50% with Turbo LoRA, FP8, and GPU Autoscaling.
Unlock AI for Your Business
To stay competitive, explore how the Normalized Transformer can transform your operations:
– **Identify Automation Opportunities:** Pinpoint customer interaction areas that can benefit from AI.
– **Define KPIs:** Measure the impact of your AI initiatives on business outcomes.
– **Select the Right AI Solution:** Choose customizable tools that fit your needs.
– **Implement Gradually:** Start small, gather data, and expand wisely.
For AI KPI management advice, reach us at hello@itinai.com. For continuous AI insights, follow us on Telegram t.me/itinainews or Twitter @itinaicom. Discover how AI can enhance your sales and customer engagement by visiting itinai.com.