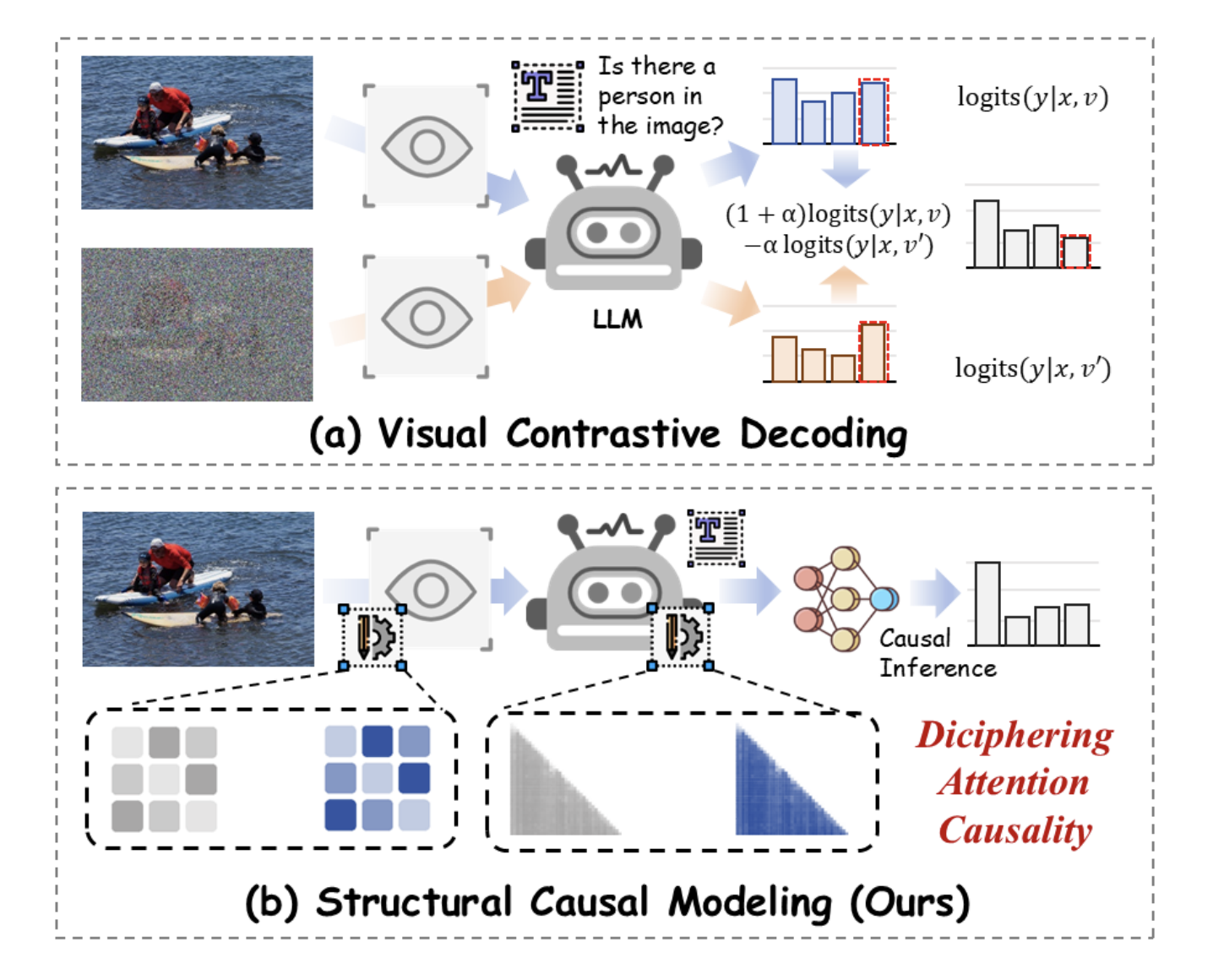
Understanding Multimodal Large Language Models (MLLMs)
Multimodal Large Language Models (MLLMs) use advanced Transformer models to process various types of data, like text and images. However, they struggle with biases in their initial setup, known as modality priors, which can lower the quality of their outputs. These biases affect the model’s attention mechanism—how it prioritizes different inputs—leading to issues such as multimodal hallucinations and reduced performance.
Recent Innovations
New MLLM models, such as VITA and Cambrian-1, have shown impressive results across multiple data types. Additionally, researchers are enhancing performance without further training through methods like VCD (Visual Contrastive Decoding) and OPERA, utilizing human insights. Strategies to tackle biases include combining visual components and creating benchmarks like VLind-Bench to assess these biases effectively.
Introducing CAUSALMM
Researchers from various universities have created CAUSALMM, a framework aimed at overcoming the challenges of modality priors in MLLMs. This framework employs a structural causal model and techniques like intervention to better understand how attention impacts outputs, even with existing biases.
Evaluation and Results
CAUSALMM has been rigorously tested against several benchmarks, including VLind-Bench, POPE, and MME, comparing its effectiveness with existing models like LLaVa-1.5 and Qwen2-VL. Key findings include:
- Significant performance gains in balancing visual and language biases.
- Improved handling of object-level hallucinations, with an average improvement of 5.37%.
- Enhanced capabilities in complex queries, like counting, across different benchmarks.
Conclusions and Future Directions
CAUSALMM offers a promising approach to addressing modality priors by treating them as confounding factors. Its innovative use of structural causal modeling and attention adjustments helps improve the quality of MLLM outputs, paving the way for more reliable multimodal intelligence in the future.
Get Involved
Check out the Paper and GitHub for more details. Follow us on Twitter, and join our Telegram Channel and LinkedIn Group for updates. If you enjoy our insights, consider subscribing to our newsletter and joining our 50k+ ML SubReddit.
Transform Your Business with AI
To stay competitive, consider leveraging CAUSALMM for your AI strategies:
- Identify Automation Opportunities: Find customer interaction points that can benefit from AI.
- Define KPIs: Ensure your AI projects have clear, measurable goals.
- Select an AI Solution: Choose tools that meet your specific needs.
- Implement Gradually: Start with pilot projects, collect data, and expand wisely.
For AI KPI management advice, connect with us at hello@itinai.com, and stay updated through our Telegram and Twitter channels.
Explore AI Solutions for Sales and Customer Engagement
Discover how AI can revolutionize your business processes by visiting itinai.com.