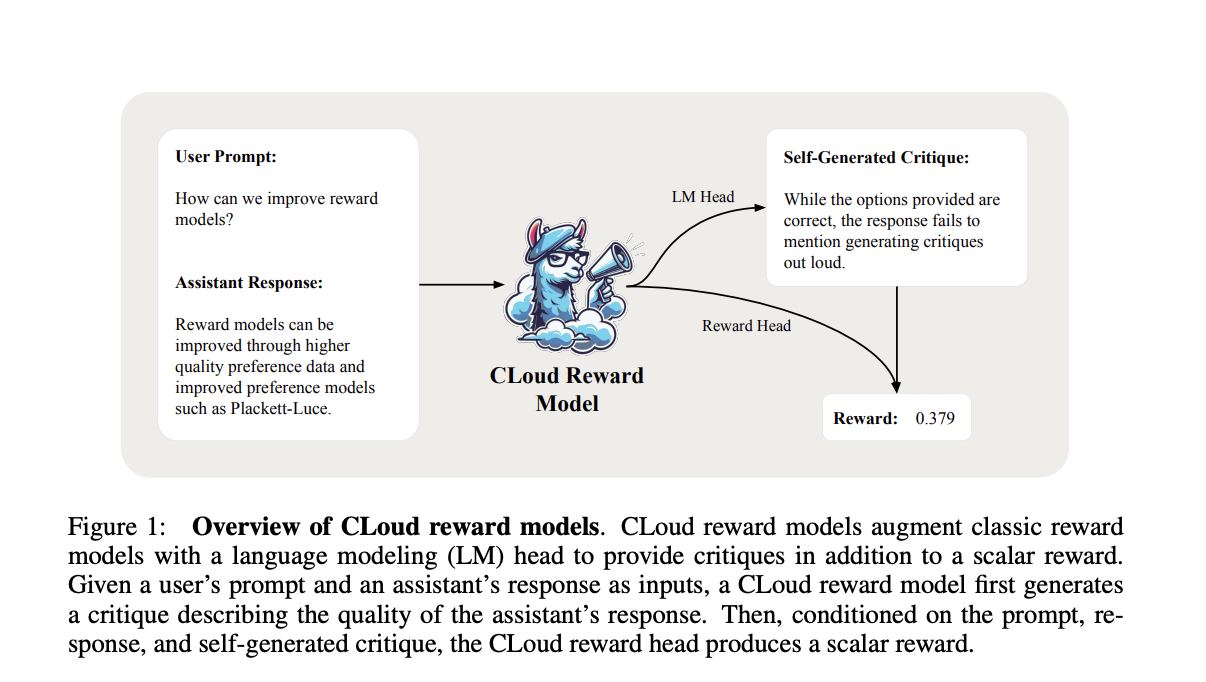
Practical Solutions for Improving RLHF with Critique-Generated Reward Models
Overview
Language models in reinforcement learning from human feedback (RLHF) face challenges in accurately capturing human preferences. Traditional reward models struggle to reason explicitly about response quality, hindering their effectiveness in guiding language model behavior. The need for a more effective method is evident.
Proposed Solutions
Researchers have introduced Critique-out-Loud (CLoud) reward models, which aim to improve language model performance in RLHF. These models generate detailed critiques of assistant responses before producing scalar rewards for response quality, combining the strengths of classic reward models and the LLM-as-a-Judge framework.
CLoud models are trained using a preference dataset and supervised fine-tuning on oracle critiques for critique generation. The training process involves exploring multi-sample inference techniques, such as self-consistency, to enhance performance.
Value and Benefits
CLoud reward models significantly outperform classic reward models in pairwise preference classification accuracy and win rates in various benchmarks. They offer superior performance in guiding language model behavior and demonstrate substantial improvements over classic reward models.
Future Opportunities
CLoud reward models establish a new paradigm for improving reward models through variable inference computing, laying the groundwork for more sophisticated and effective preference modeling in language model development.
AI Integration for Business
Discover how AI can redefine your way of work and sales processes. Identify automation opportunities, define KPIs, select AI solutions, and implement gradually to stay competitive and evolve your company with AI.
Contact Us
For AI KPI management advice and continuous insights into leveraging AI, connect with us at hello@itinai.com or stay tuned on our Telegram t.me/itinainews or Twitter @itinaicom.