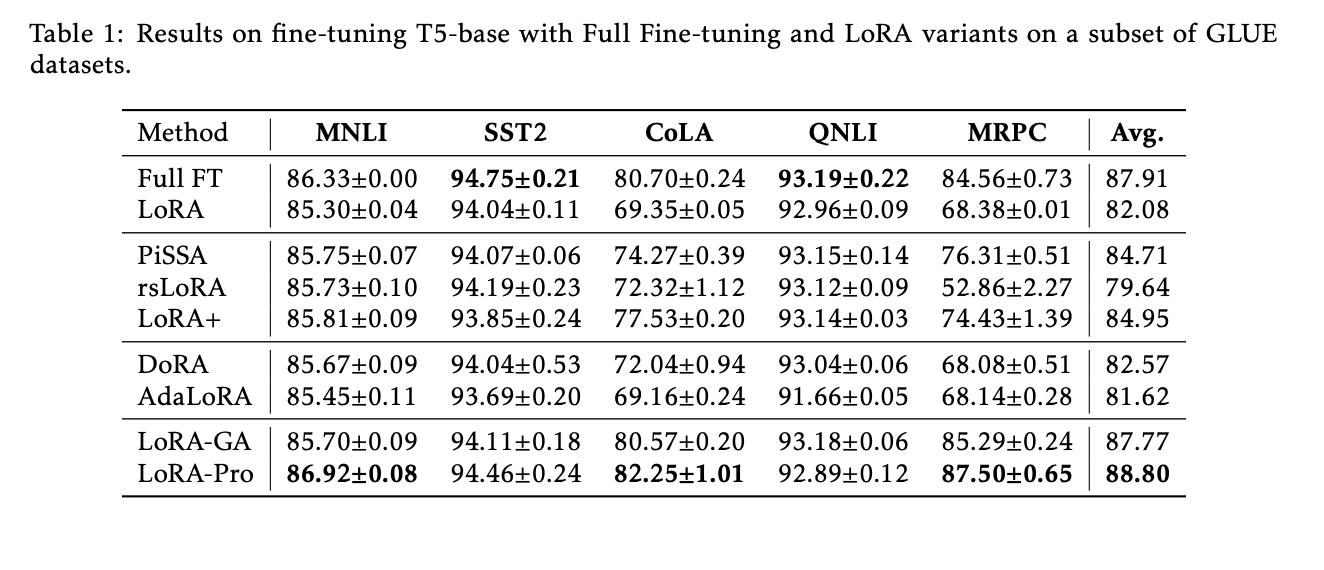
Practical Solutions for Parameter-Efficient Fine-Tuning in Machine Learning
Introduction
Parameter-efficient fine-tuning methods are essential for adapting large machine learning models to new tasks. These methods aim to make the adaptation process more efficient and accessible, especially for deploying large foundational models constrained by high computational costs and extensive parameter counts.
Challenges and Advances
The core challenge addressed in recent research is the performance gap between low-rank adaptation methods like LoRA and full fine-tuning. To bridge this gap, researchers have introduced LoRA-Pro, a novel method that enhances the optimization process by introducing the concept of “Equivalent Gradient.” This allows LoRA-Pro to closely mimic the optimization dynamics of full fine-tuning, thus improving the performance of LoRA.
Experimental Validation
Extensive experiments on natural language processing tasks have demonstrated the effectiveness of LoRA-Pro. It outperformed standard LoRA, achieving the highest scores on three out of five datasets, with average scores surpassing standard LoRA by a margin of 6.72%. These results underscore the capability of LoRA-Pro to narrow the performance gap with full fine-tuning, making it a significant improvement over existing parameter-efficient fine-tuning methods.
Value and Practical Implementation
LoRA-Pro provides a valuable tool for deploying large foundational models in a more resource-efficient manner by maintaining the efficiency of LoRA and achieving performance levels closer to full fine-tuning.
AI Implementation Tips
If you’re looking to evolve your company with AI, consider the following tips:
- Identify Automation Opportunities
- Define KPIs
- Select an AI Solution
- Implement Gradually
For AI KPI management advice, connect with us at hello@itinai.com. Stay tuned on our Telegram channel or Twitter for continuous insights into leveraging AI.
Discover how AI can redefine your sales processes and customer engagement. Explore solutions at itinai.com.