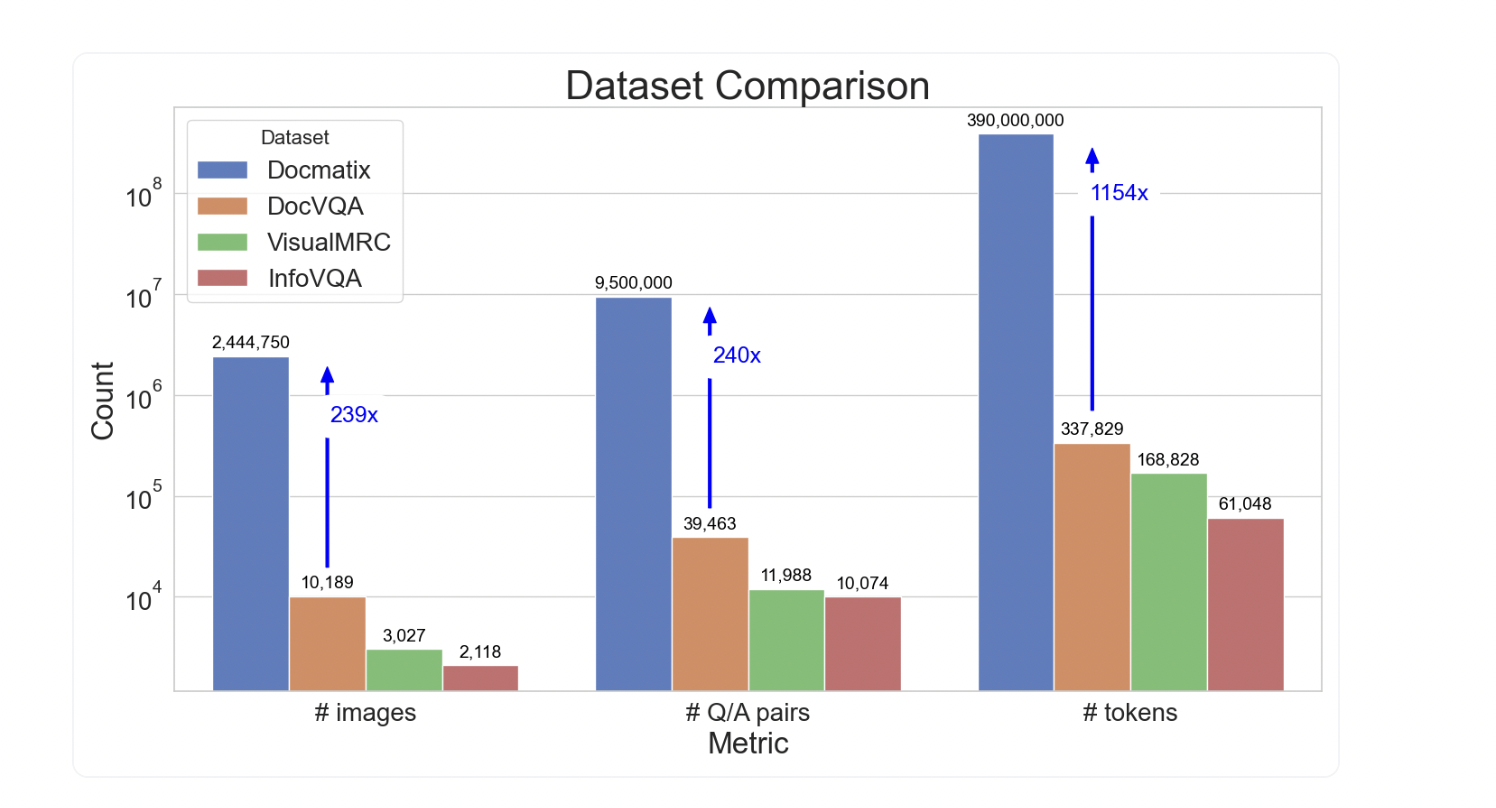
Practical Solutions and Value of Docmatix: A Dataset for Document Visual Question Answering
Challenges in DocVQA
Document Visual Question Answering (DocVQA) faces challenges due to the complexity of collecting and annotating data from various document formats. Domain-specific differences, privacy concerns, and the lack of document-structure uniformity further complicate dataset development.
Importance of DocVQA Datasets
Despite the challenges, the need for DocVQA datasets is crucial for enhancing model performance, benchmarking, and automating document-related processes across sectors.
Introduction of Docmatix
The new monumental Docmatix dataset contains 2.4 million pictures and 9.5 million Q/A pairs, extracted from 1.3 million PDF documents. This significant scale showcases the potential impact of Docmatix on document accessibility.
Creation and Validation of Docmatix
The researchers used a Phi-3-small model to create Q/A pairs from the PDFA transcriptions and validated the dataset by removing hallucinated Q/A pairings. The processed images are now easily accessible to users, ensuring the dataset’s reliability.
Improving Model Performance with Docmatix
The researchers conducted ablation experiments to fine-tune the prompts and assess Docmatix’s performance. Training on a small subset of Docmatix showed a relative improvement of about 20% in model performance, highlighting the dataset’s potential impact on model training.
Future of DocVQA Models
The team encourages the open-source community to use Docmatix to reduce the disparity between proprietary and open-sourced Vision-Language Models (VLMs) and to train new, high-performing DocVQA models.
AI Solutions to Redefine Work and Sales Processes
AI can redefine work by automating processes and redefine sales processes and customer engagement. To leverage AI for your business, connect with us at hello@itinai.com and stay tuned for continuous insights into leveraging AI.