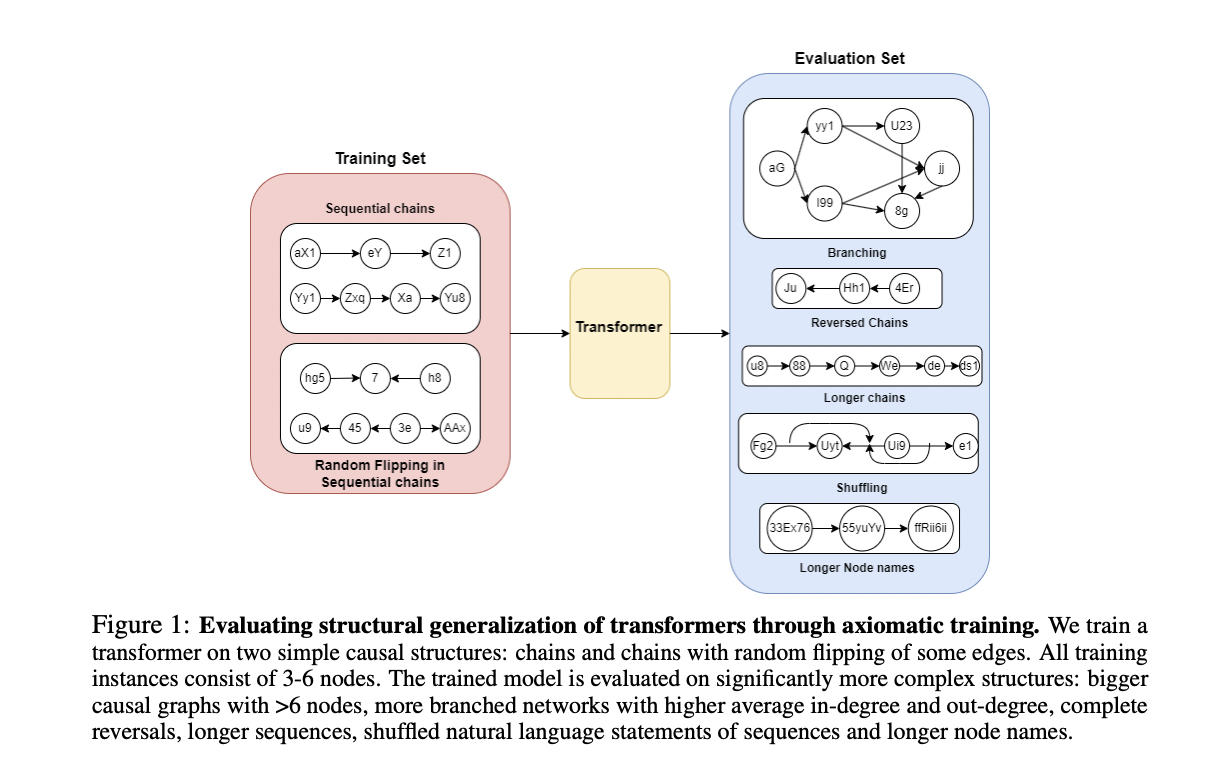
Enhancing AI Models with Axiomatic Training for Causal Reasoning
Revolutionizing Causal Reasoning in AI
Artificial intelligence (AI) has made significant strides in traditional research, but faces challenges in causal reasoning. Training AI models to understand cause-and-effect relationships using accessible data sources is crucial for their efficiency and accuracy.
Challenges in Existing AI Models
Current AI models heavily rely on large datasets with explicit or inferred causal relationships, limiting their ability to generalize across different scenarios.
Introducing Axiomatic Training
Researchers have introduced axiomatic training, a novel approach that involves training models on multiple demonstrations of causal axioms or rules. This method enhances the models’ ability to generalize causal reasoning to new and complex scenarios, shifting the focus from data-intensive training to a principle-based approach.
Remarkable Results
The axiomatic training approach has shown remarkable results, with AI models demonstrating exceptional generalization capabilities, outperforming larger models in specific tests and achieving high accuracy rates for complex causal chains.
Implications and Future Directions
Axiomatic training offers a more efficient and scalable approach to teaching causal reasoning, potentially transforming how AI systems are trained for causal inference tasks. This success indicates a promising direction for future research and applications in AI.
Evolve Your Company with AI
Discover how AI can redefine your way of work and sales processes, and identify automation opportunities to stay competitive and enhance customer engagement.
For AI KPI management advice, connect with us at hello@itinai.com. For continuous insights into leveraging AI, stay tuned on our Telegram channel or Twitter.