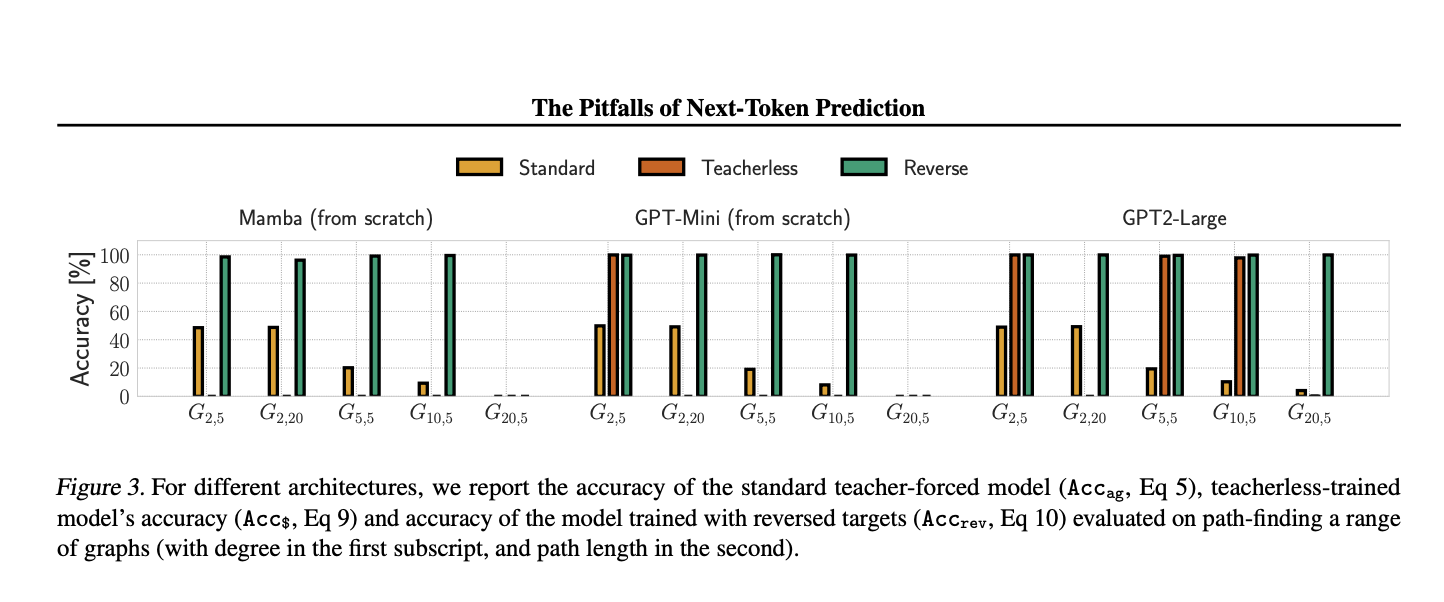
The Pitfalls of Next-Token Prediction
Challenges in Artificial Intelligence
One of the emerging challenges in artificial intelligence is whether next-token prediction can truly model human intelligence, particularly in planning and reasoning. Despite its extensive application in modern language models, this method might be inherently limited when it comes to tasks that require advanced foresight and decision-making capabilities. This challenge is significant as overcoming it could enable the development of AI systems capable of more complex, human-like reasoning and planning, thus expanding their utility in various real-world scenarios.
Current Methods and Limitations
Current methods, primarily relying on next-token prediction through autoregressive inference and teacher-forcing during training, have been successful in many applications, such as language modeling and text generation. However, these methods face significant limitations. Autoregressive inference suffers from the compounding of errors, where even minor inaccuracies in predictions can snowball, leading to substantial deviations from the intended sequence over long outputs. Teacher-forcing, on the other hand, fails to accurately learn next-token prediction in certain tasks, inducing shortcuts and hindering effective planning and reasoning.
Novel Approach: Multi-Token Prediction
The researchers introduce a novel approach by advocating for a multi-token prediction objective, which aims to address the shortcomings of existing next-token prediction methods. This approach proposes predicting multiple tokens in advance rather than relying solely on sequential next-token predictions. By doing so, it mitigates the issues arising from error compounding in autoregressive inference and the shortcut learning in teacher-forcing, offering a more robust and accurate method for sequence prediction, enhancing the model’s ability to plan and reason over longer sequences.
Empirical Evaluation
The proposed method involves predicting multiple tokens at once during training, thus avoiding the pitfalls of traditional teacher-forcing and autoregressive methods. The researchers designed a minimal planning task using a path-finding problem on a graph to empirically demonstrate the failure of traditional methods. Both the Transformer and Mamba architectures were tested, revealing that these models fail to learn the task accurately under traditional next-token prediction methods.
Impact and Conclusion
The findings show that the proposed multi-token prediction approach demonstrated a significant improvement in accuracy and performance, successfully mitigating the issues seen with autoregressive inference and teacher-forcing. This method represents a significant advancement in AI research, offering a more robust and accurate method for sequence prediction. The contribution lies in highlighting the limitations of current methods and providing a promising alternative that enhances the planning and reasoning capabilities of AI models.
Check out the Paper. All credit for this research goes to the researchers of this project.
AI Solutions for Business
If you want to evolve your company with AI, stay competitive, use for your advantage Beyond Next-Token Prediction: Overcoming AI’s Foresight and Decision-Making Limits. Discover how AI can redefine your way of work. Identify Automation Opportunities, Define KPIs, Select an AI Solution, Implement Gradually. For AI KPI management advice, connect with us at hello@itinai.com.
Discover how AI can redefine your sales processes and customer engagement. Explore solutions at itinai.com.
Also, don’t forget to follow us on Twitter.
Join our Telegram Channel and LinkedIn Group.
If you like our work, you will love our newsletter.
Don’t Forget to join our 46k+ ML SubReddit.