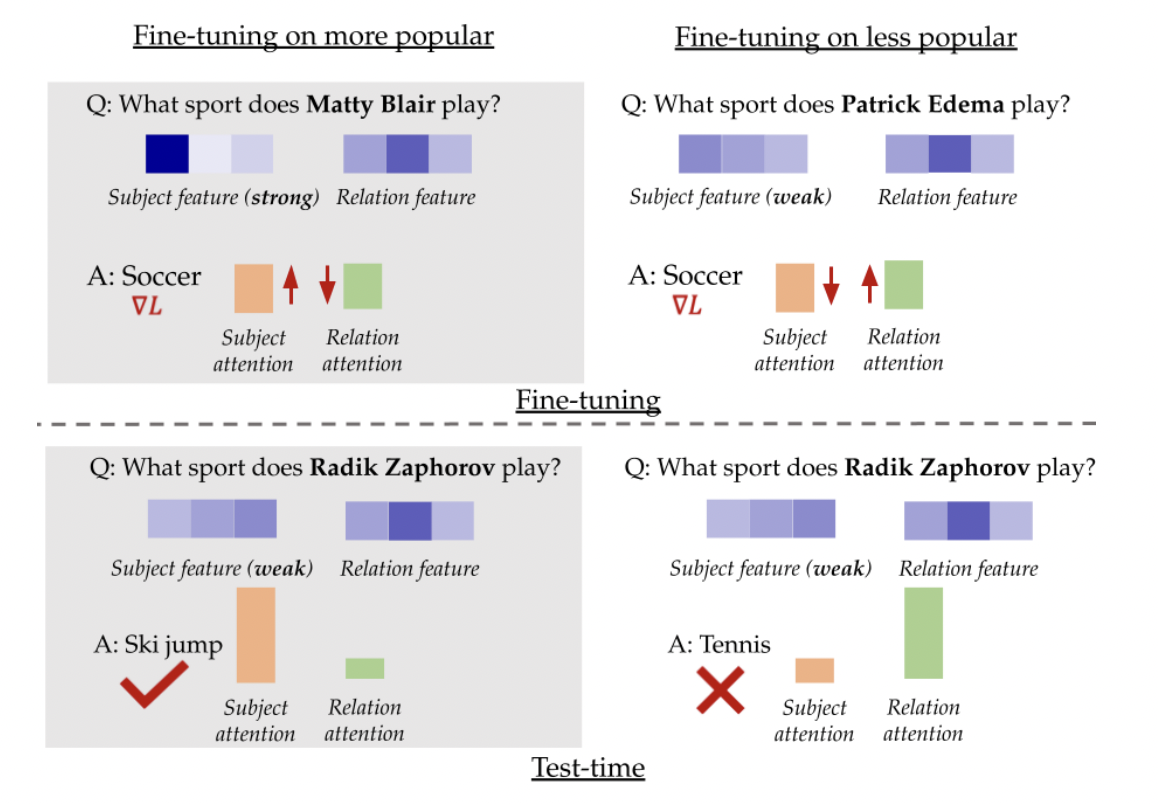
Practical Solutions for Enhancing Language Model Accuracy
Challenges in Language Model Factuality
Large language models (LLMs) are powerful but may produce incorrect responses, posing challenges for knowledge-based applications.
Approaches to Improve Factuality
Researchers are exploring techniques such as manipulating attention mechanisms, using unsupervised internal probes, and developing methods for LLMs to abstain from answering uncertain questions.
Insights from Research
Research from Carnegie Mellon University and Stanford University shows that fine-tuning LLMs on well-encoded facts significantly improves factuality, providing a new perspective on enhancing LLM accuracy.
Impact of Fine-Tuning Data
Experimental results across multiple datasets and models consistently show that fine-tuning on well-known facts enhances overall factuality, challenging conventional approaches to QA dataset design.
Practical AI Solutions
Companies can use AI to redefine their work processes, identify automation opportunities, define KPIs, select AI solutions, and implement AI gradually for business impact.
Connect with Us
For AI KPI management advice and continuous insights into leveraging AI, connect with us at hello@itinai.com or follow us on Telegram and Twitter.