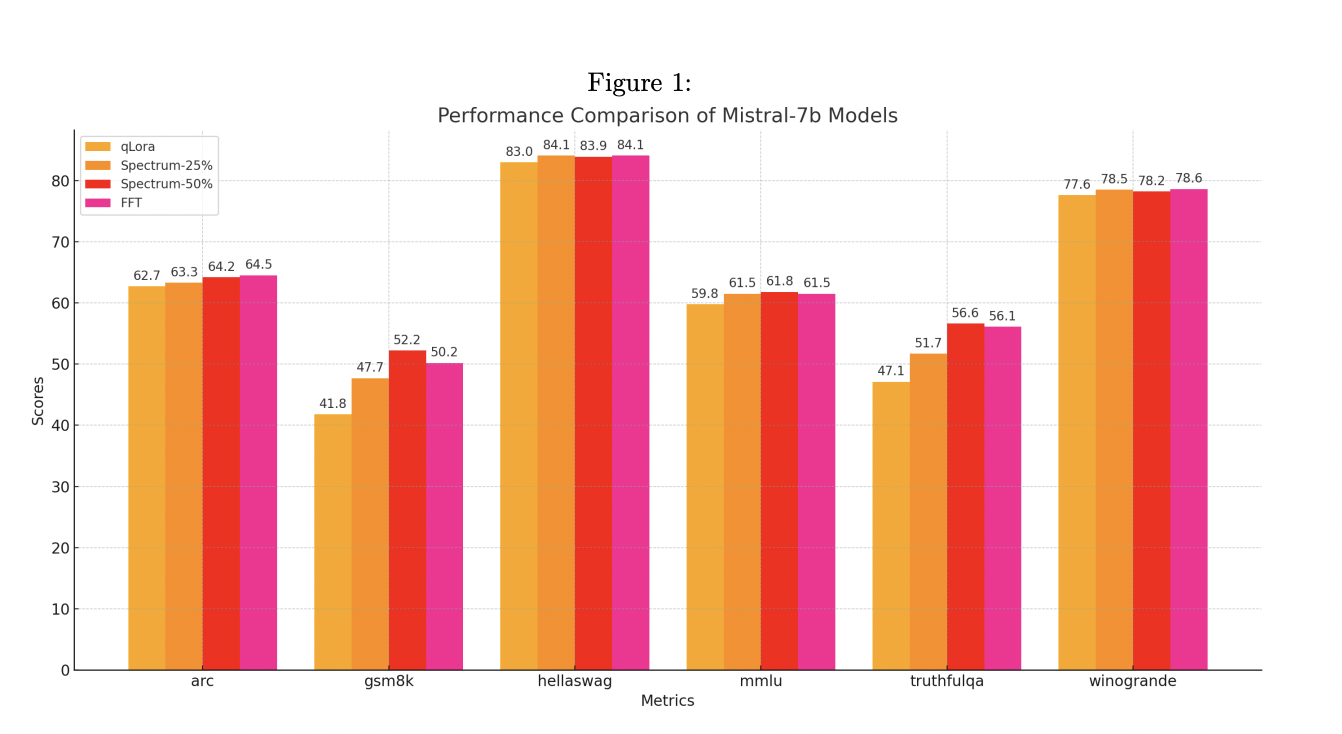
Practical Solutions for Efficient LLM Training
Challenges in Large Language Model Training
Large language models (LLMs) require significant computational resources and time for training, posing challenges for researchers and developers. Efficient training without compromising performance is crucial.
Novel Methods for Efficient Training
Methods like QLoRA and LASER reduce memory usage and improve model performance, while Spectrum targets specific layers based on their signal-to-noise ratio (SNR), significantly reducing GPU memory usage and maintaining high performance.
Methodology and Experiment Results
Spectrum’s methodology is grounded in Random Matrix Theory and Marchenko-Pastur distribution, enabling precise targeting of informative layers. Experimental results show competitive performance and significant reductions in memory usage and training time.
Efficiency in Large-Scale Model Training
Spectrum’s efficiency is evident in distributed training environments, achieving significant memory savings per GPU. Combining Spectrum with QLoRA further enhances memory efficiency and training speed.
Impact and Future Potential
Spectrum offers a groundbreaking approach to train large language models efficiently, holding potential for democratizing LLM research and enabling broader applications in various fields.
AI Solutions for Business Advancement
Identify automation opportunities, define KPIs, select AI solutions, and implement gradually to evolve your company with AI. For AI KPI management advice and continuous insights, connect with us at hello@itinai.com or stay tuned on our Telegram or Twitter.
AI for Sales Processes and Customer Engagement
Discover how AI can redefine your sales processes and customer engagement. Explore solutions at itinai.com.