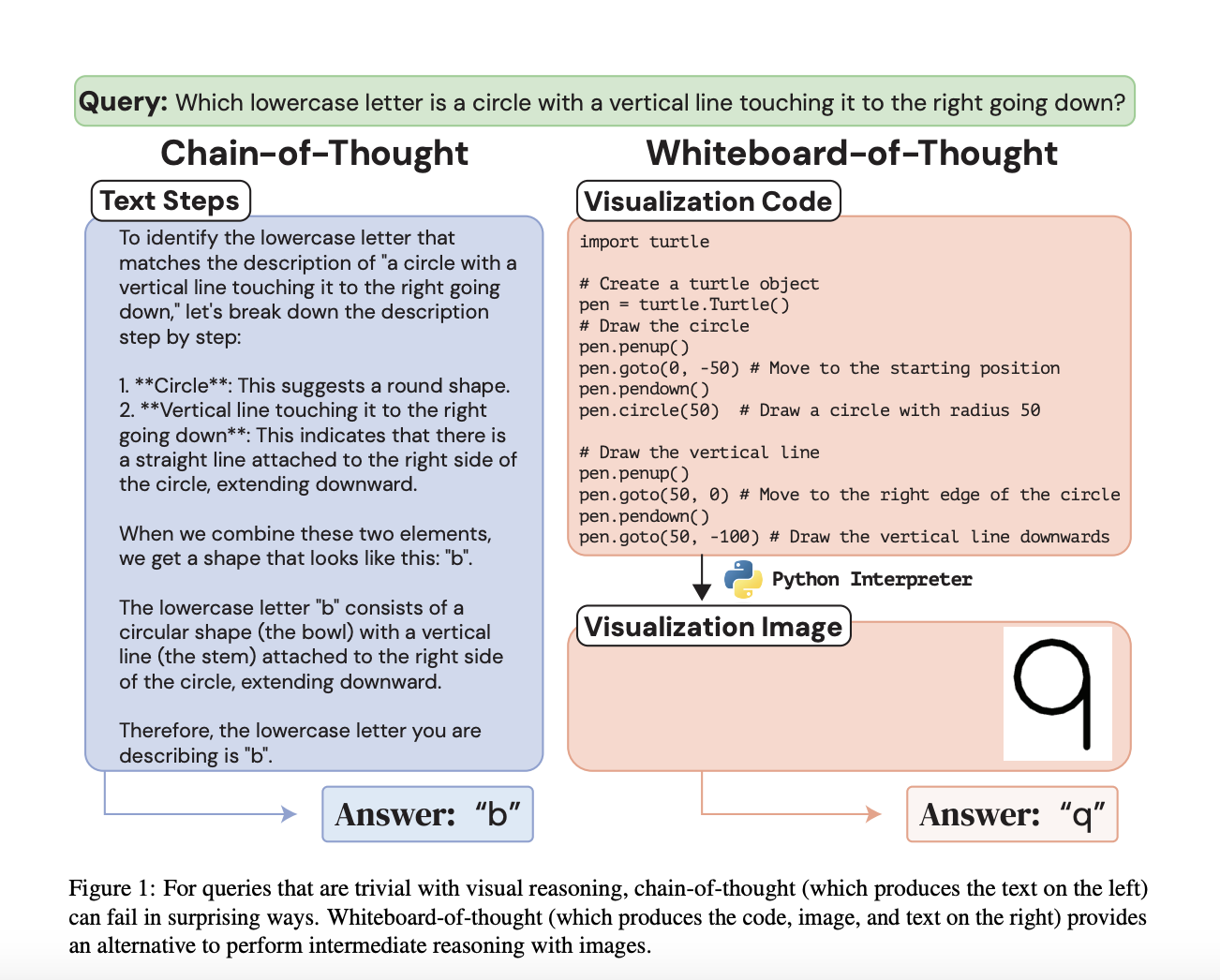
Practical Solutions for Enhancing Visual Reasoning Abilities of AI Models
Introduction
Large language models (LLMs) have revolutionized natural language processing (NLP) by leveraging increased parameters and training data for various reasoning tasks. However, they struggle with visual and spatial reasoning. To address these limitations, researchers have introduced the Whiteboard-of-Thought (WoT) prompting method to enhance the visual reasoning abilities of multimodal large language models (MLLMs).
Key Approaches
Existing approaches include Intermediate Reasoning for Language Models, Tool Usage and Code Augmentation, and Visual and Spatial Reasoning in LLMs and MLLMs. The WoT prompting method allows MLLMs to draw out reasoning steps as images, enabling state-of-the-art results on difficult natural language tasks requiring visual and spatial reasoning.
Value and Applications
WoT enables MLLMs to create and process images to improve query responses. It addresses the limitations of current MLLMs in producing visual outputs and achieves superior accuracy compared to traditional methods. The approach also eliminates dependencies on 2D-grid-specific textual knowledge, making it applicable across various geometries.
Conclusion and Next Steps
WoT presents a zero-shot method for visual reasoning across modalities in MLLMs. Future research aims to enhance MLLMs’ understanding of detailed geometric figures. To evolve your company with AI and stay competitive, consider leveraging WoT to enhance visual reasoning abilities of MLLMs.
AI Solutions for Your Business
Discover how AI can redefine your way of work by identifying automation opportunities, defining KPIs, selecting AI solutions, and implementing them gradually. For AI KPI management advice and insights into leveraging AI, connect with us at hello@itinai.com or stay tuned on our Telegram t.me/itinainews or Twitter @itinaicom.
Discover how AI can redefine your sales processes and customer engagement. Explore solutions at itinai.com.