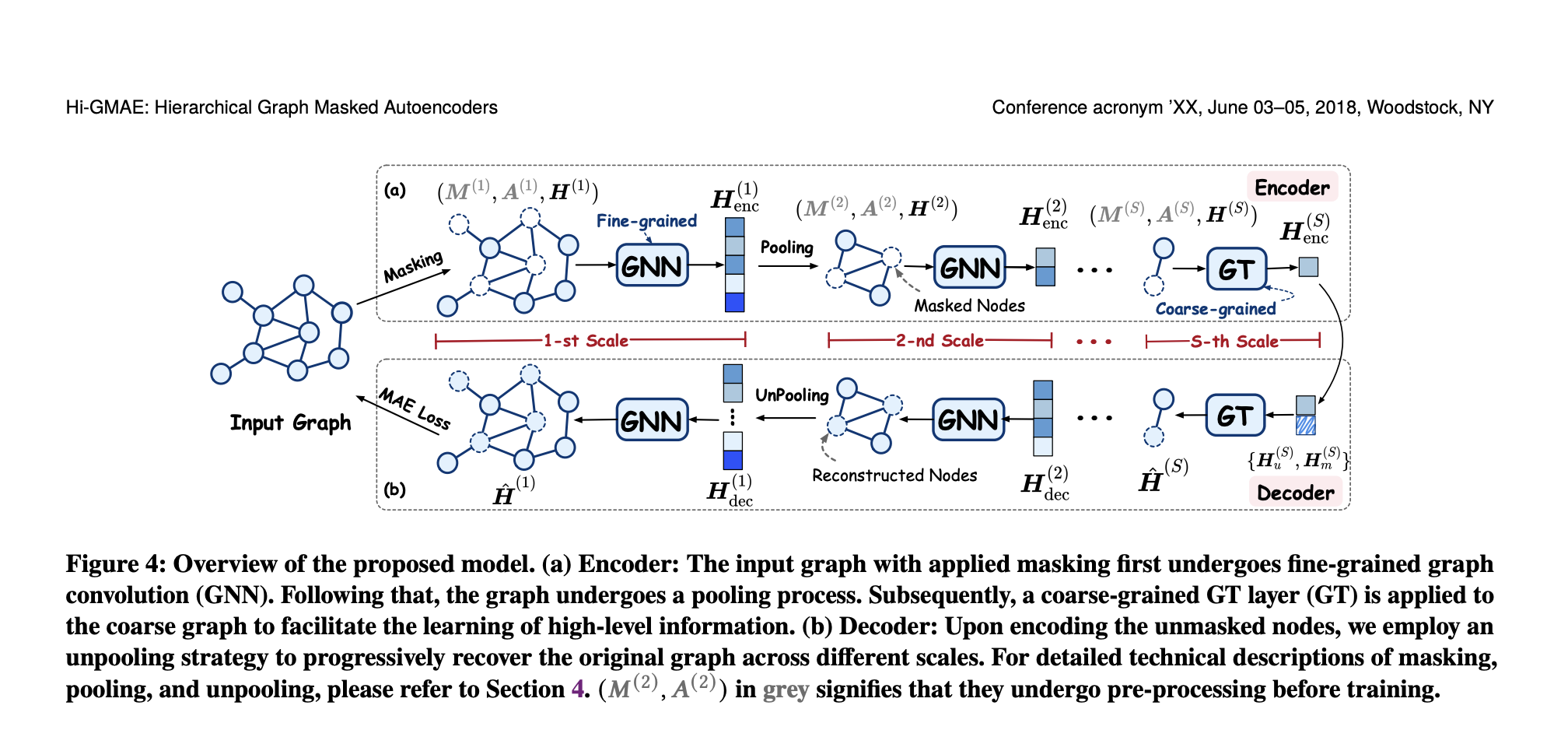
Graph Self-supervised Pre-training (GSP) Techniques
In graph analysis, labeled data poses a challenge for traditional supervised learning methods. Graph Self-supervised Pre-training (GSP) techniques have emerged to overcome this limitation by extracting meaningful representations from graph data without the need for labeled examples.
Contrastive and Generative GSP Methods
GSP methods are broadly classified into two categories: contrastive and generative. Contrastive methods create multiple graph views through augmentation and learn representations by contrasting positive and negative samples. Generative methods focus on learning node representations via a reconstruction objective.
Hierarchical Graph Masked AutoEncoders (Hi-GMAE)
Hi-GMAE is a framework designed to capture hierarchical information in graphs. It comprises three main components: multi-scale coarsening, Coarse-to-Fine (CoFi) masking with recovery, and Fine- and Coarse-Grained (Fi-Co) encoder and decoder.
Validation and Performance
Extensive experiments demonstrated that Hi-GMAE outperforms existing state-of-the-art models in contrastive and generative pre-training domains, highlighting its superior capability in capturing and leveraging hierarchical graph information.
Practical AI Solutions
Identify Automation Opportunities, Define KPIs, Select an AI Solution, and Implement Gradually. Connect with us at hello@itinai.com for AI KPI management advice and stay tuned on our Telegram t.me/itinainews or Twitter @itinaicom for continuous insights into leveraging AI.
AI Sales Bot
Consider the AI Sales Bot from itinai.com/aisalesbot designed to automate customer engagement 24/7 and manage interactions across all customer journey stages.