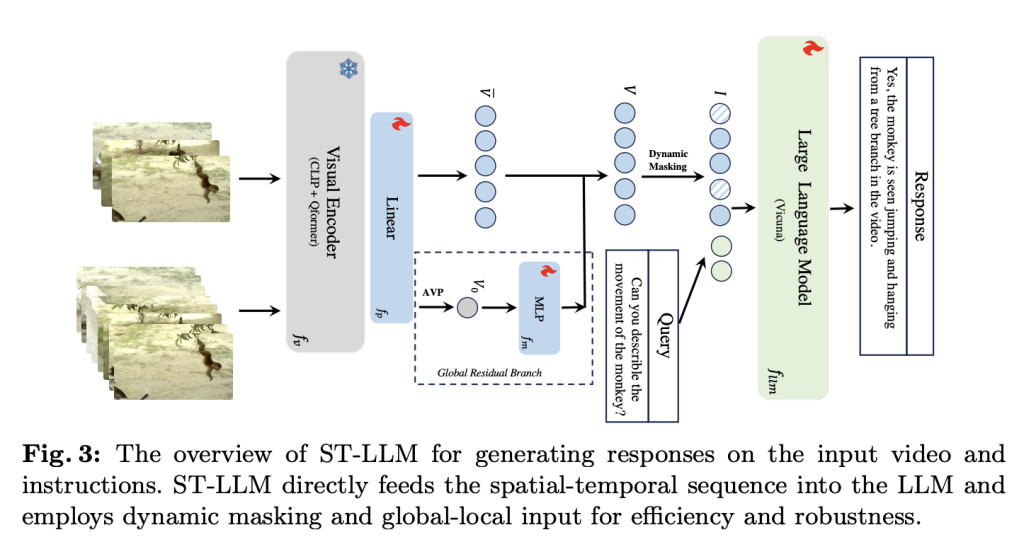
The Impact of ST-LLM in Video Understanding
Introduction
The world of artificial intelligence has seen remarkable advancements in Large Language Models (LLMs) like GPT, PaLM, and LLaMA, showcasing their potential for natural language understanding and generation. However, extending their capabilities to videos with rich temporal information has been a challenge.
The Challenge
Existing methods for video understanding in LLMs have limitations, such as ineffective capturing of dynamic temporal sequences and demanding extensive computational resources.
The Solution: ST-LLM
A team of researchers from Peking University and Tencent proposed ST-LLM, leveraging LLMs to process raw spatial-temporal video tokens directly. This approach addresses the limitations of existing methods and enhances the model’s robustness to varying video lengths during inference.
Key Features of ST-LLM
– ST-LLM feeds all video frames into the LLM, effectively modeling spatial-temporal sequences.
– It introduces a dynamic video token masking strategy and masked video modeling during training.
– For long videos, it employs a unique global-local input mechanism, preserving the modeling of video tokens within the LLM.
Effectiveness of ST-LLM
Extensive experiments have demonstrated the remarkable effectiveness of ST-LLM, showcasing superior temporal understanding and state-of-the-art performance in various video benchmarks.
Practical AI Solutions
To evolve your company with AI, consider using ST-LLM for video understanding. Additionally, explore practical AI solutions like the AI Sales Bot from itinai.com/aisalesbot, designed to automate customer engagement and manage interactions across all customer journey stages.
For more information and insights into leveraging AI, connect with us at hello@itinai.com or stay tuned on our Telegram t.me/itinainews or Twitter @itinaicom.