The text provides an introduction to Simple Linear Regression in Machine Learning. It emphasizes the basic concepts, mathematical computation, optimization methods (OLS and Gradient Descent), model evaluation using R² and RMSE, and key assumptions for successful application. The author invites readers to stay tuned for an end-to-end project demonstration in the upcoming piece.
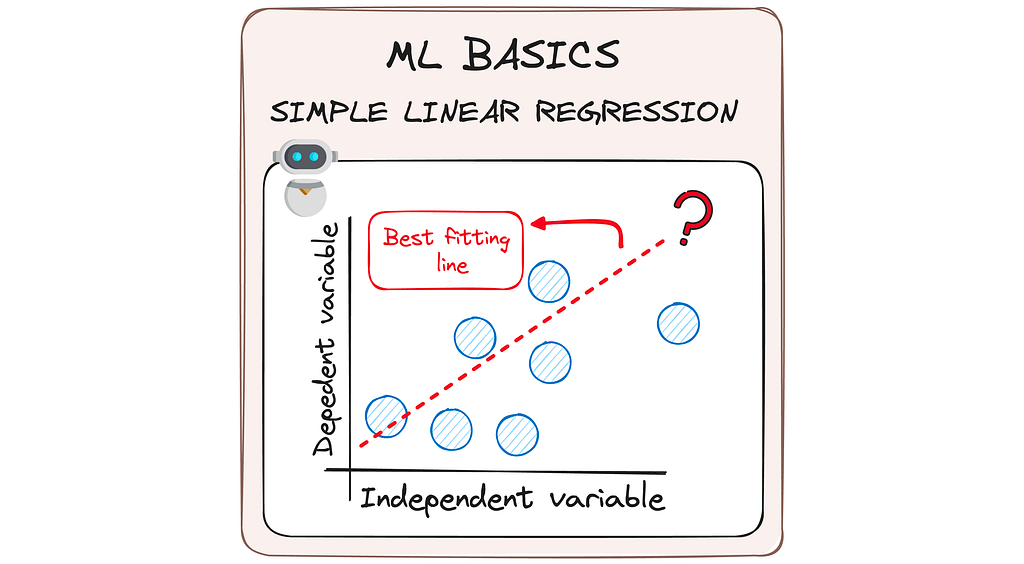
“`html
MLBasics — Simple Linear Regression
Demystifying Machine Learning Algorithms with The Simplicity of Linear Regression
In the world of data and computer programs, the concept of Machine Learning might sound like a tough nut to crack, full of tricky math and complex ideas.
This is why today I want to slow down and check out the basic stuff that makes all this work. I’m kicking off a fresh set of articles I’m calling MLBasics.
We’re going to revisit the simple, yet super-important, models that are the ABCs of ML. Think of it as starting with the easy pieces of a big puzzle. We’re going back to the simple stuff, where it’s easy to get what’s going on.
So come along for the ride as we break it down and make it all clear.
Let’s dive into Simple Linear Regression, step by step, together! 👇🏻🤓
1. Introduction to the Simple Linear Regression
The realm of predictive analysis is vast, yet at its heart lies Linear Regression — the simplest method to make sense of data trends.
…
6. Assumptions to apply linear regression
For Linear Regression to work well, there are a few key things we need to keep in mind before applying it to a random dataset.
…
Wrapping Up
In our MLBasics journey, we’ve started demystifying Simple Linear Regression, showcasing its fundamental role in understanding data trends.
We’ve explored the relationship between independent and dependent variables, emphasizing error minimization for accurate predictions.
Our discussion included mathematical approaches like Ordinary Least Squares (OLS) and Gradient Descent for optimizing the model.
We evaluated the model’s effectiveness using R² and RMSE metrics and stressed the importance of meeting key assumptions for successful application.
Stay tuned for our next piece, where we’ll bring theory to practice in an end-to-end project, demonstrating Simple Linear Regression.
You can subscribe to my Medium Newsletter to stay tuned and receive my content. I promise it will be unique!
You can find me on Twitter and LinkedIn as well!
AI Solutions for Middle Managers
If you want to evolve your company with AI, stay competitive, use for your advantage MLBasics — Simple Linear Regression | by Josep Ferrer | Medium.
Discover how AI can redefine your way of work. Identify Automation Opportunities: Locate key customer interaction points that can benefit from AI.
Define KPIs: Ensure your AI endeavors have measurable impacts on business outcomes.
Select an AI Solution: Choose tools that align with your needs and provide customization.
Implement Gradually: Start with a pilot, gather data, and expand AI usage judiciously.
For AI KPI management advice, connect with us at hello@itinai.com. And for continuous insights into leveraging AI, stay tuned on our Telegram t.me/itinainews or Twitter @itinaicom.
Spotlight on a Practical AI Solution:
Consider the AI Sales Bot from itinai.com/aisalesbot designed to automate customer engagement 24/7 and manage interactions across all customer journey stages.
Discover how AI can redefine your sales processes and customer engagement. Explore solutions at itinai.com.
“`