The text discusses the challenges and potential of generative AI (GenAI) in driving business value. It highlights the importance of developing differentiated and valuable features, addressing data, technological, and infrastructure challenges, and involving key players like data engineers. It emphasizes the need for a strategic approach to leverage GenAI effectively in business.
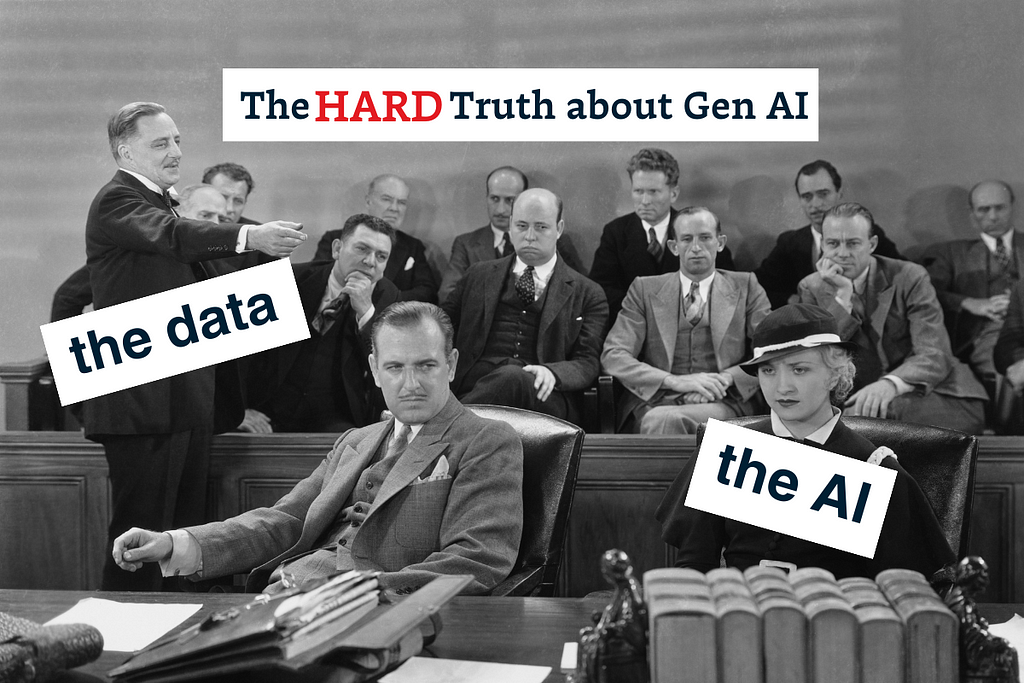
“`html
5 Hard Truths About Generative AI for Middle Managers
Introduction
GenAI is everywhere, and organizations are feeling the pressure to leverage its benefits. However, building a generative AI model that drives real business value is a challenging task. Here are 5 hard truths about generative AI for middle managers and practical solutions to address them.
Hard Truth #1: Adoption and Monetization Challenges
Many AI initiatives lack well-defined user problems and struggle with low adoption rates. To address this, it’s crucial to focus on connecting generative AI with proprietary data and business context to drive differentiated value. A RAG model is essential for providing access to enterprise proprietary data, leading to better adoption and monetization.
Hard Truth #2: Fear of Embracing Generative AI
Generative AI can be intimidating due to potential risks such as data mishandling and legal repercussions. However, it’s essential to understand the data being fed into GenAI and ensure its accuracy. Waiting for the hype to die down is not a viable strategy, as it can lead to missed opportunities and business disruption.
Hard Truth #3: Complexity of RAG
Retrieval augmented generation (RAG) and fine tuning are crucial for the future of enterprise generative AI. While RAG is a simpler approach, it still presents complexities in terms of development and architecture. However, RAG offers the benefit of grounding LLMs in accurate proprietary data, making it more valuable.
Hard Truth #4: Data Readiness Challenges
Even with a perfect RAG pipeline and fine-tuned model, organizations may still lack clean, well-modeled datasets to plug into their AI initiatives. Establishing useful, reliable, and consolidated datasets in a modern data platform is essential for GenAI readiness.
Hard Truth #5: Exclusion of Critical Players
Many data teams overlook the importance of including data engineers in their GenAI initiatives. Data engineers play a critical role in understanding proprietary business data and building pipelines to make that data available to LLMs via RAG. Their involvement is essential for the success of GenAI initiatives.
Practical AI Solutions
To address these hard truths and leverage generative AI for competitive advantage, middle managers can:
- Identify Automation Opportunities
- Define KPIs for AI Impact
- Select Customizable AI Solutions
- Implement AI Gradually
For AI KPI management advice and insights into leveraging AI, connect with us at hello@itinai.com. Explore AI solutions at itinai.com/aisalesbot to redefine your sales processes and customer engagement.
“`