The text explores the obstacles faced by data teams in achieving tangible Return on Investment (ROI). It outlines steps for measuring ROI, such as establishing key performance indicators, improving them through data, and measuring the data’s impact. The article identifies various obstacles, including alignment with business priorities, setting realistic expectations, root cause analysis, taking action on insights, and measuring impact.
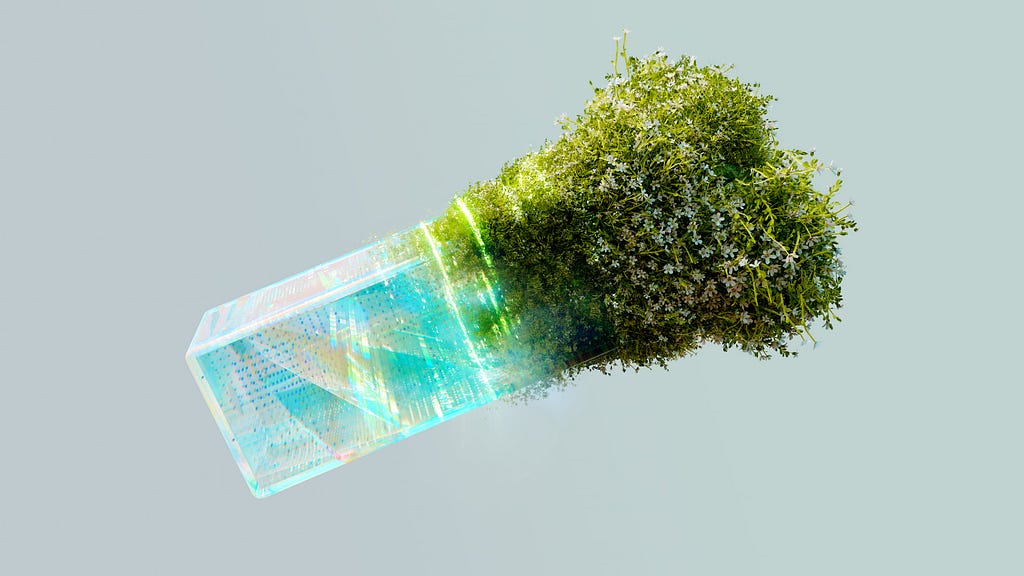
Identifying the Popular Obstacles of Data Teams in Delivering Tangible ROI
Can data leaders harvest the fruit of their labor: tangible ROI? (Image by Google Deep Mind — Pexels.com)
Return on Investment (ROI) is a profitability metric used to evaluate how well an investment has performed (Investopedia.com).
ROI is a simple term, isn’t it? To measure ROI for data, we just need to follow these 3 steps:
1. Have a set of KPIs that represent “profitability” of data
2. Improve the KPIs through data
3. Measure the impact of data’s contribution
Yet, data teams are facing obstacles in every step of this journey. Gartner’s 2023 CDO survey shows 69% of D&A leaders are struggling to deliver measurable ROI (Subhash Kari — EVP & Chief Innovation Officer at Infocepts). The inability to deliver tangible ROI is a common, and fatal, weakness of many data teams.
Despite its popularity, we do not have to see the delivery of tangible data ROI as a complex struggle. I think complex problems can be made simpler when we start from the basic and use First Principles for reasoning.
1. Have KPI that represent “profitability” of data
“Profitability” is measured in terms of tangible business impact that data can create. They are business-facing KPIs.
Obstacle 1: Access the boardroom for the right business-facing KPIs
Data leaders cannot come up with these KPIs without understanding the priorities in the boardroom. When their KPIs are the same as the boardroom’s priority, data leaders attract more attention. They will be given more room to speak, more room to probe, more room to showcase. This will create a virtuous cycle to help data leaders do a better, more relevant job, while driving more trust and gathering more investment into data.
CDOs who have business-facing KPIs and multiple business partners are 1.7 times more likely to be effective at consistently producing clear business value (Gartner, 2021)
The best way to align data KPIs with the board‘s priorities is for data leaders to be in the boardroom themselves. This leads to the importance of the CDO role. CDO have direct access to the boardroom and can identify the areas that need help the most. However, only 27 percent of the world’s largest 2,500 publicly listed firms have a CDO (Statista, 2022).
For companies without a CDO, their most senior data leaders are often bounded by functional responsibilities. A Head of Reporting’s purpose is often to support financial and MI reporting. The Head of Data Analytics often reports to the Head or a core business function. These data leaders sit far away from the C-suites and have challenges understanding the priorities in the board room. With the exceptions of data leaders whose mission is broadcasted directly from the board(a top down approach), data leaders who are a couple grades below the C-Suites are set-up to focus only on the tactical goals while losing sight of the strategic business-facing KPIs.
2. Contribute to improving the KPIs
Improving the business-facing KPIs is, first and foremost, the job of business functions. Therefore, it is crucial for data leaders to walk side-by-side with business end-users through the problem solving process and understand how data can assist them. Ironically, if a data team often refer to business as “the business”, it is very likely that silos exist between the two teams. This leads us to the next obstacle:
Obstacle 2: Setting the right expectation for data
The dangerous expectation that both data leaders and business leaders may have is for data to make magic, all by itself. This leads to disappointment down the line, when data projects do not return ROI.
Many times, the best data projects are not driven by data team, but by data-savvy people in functional teams. Marketing is the best at optimizing click-through and conversion rate, saving advertising dollars. Finance is the best at knowing which activities drive the most cost, or which products are most profitable. If there is a data team separated from the business, they exist not to replace business function in problem- solving and decision-making, but to assist them with more and better data, while maximizing the value of data-activating resources.
Obstacle 3: Identify what drive the KPIs
The analysis undertaken to improve business-facing KPIs should be, as a minimum, a combination of descriptive and diagnostic. Besides defining the problem, we also need to identify the root causes and solve the problems.
Descriptive analysis is great at defining the problem. It allows us to study the KPIs from multiple perspective. However, it falls short at pointing out the root causes. To deliver insights, what we should look for during and after descriptive analysis is forming hypotheses with diagnostic analysis. Yet, lots of Data & Analytics is hypothesis-free data mining (Roger Martin).
One solution for the lack of hypothesis is to assess whether the data is being analyzed by the right people. The ideal person is someone who can translate the data into concrete business events, form hypotheses about causal relationships between business entities, and follow an analytical method to test their hypothesis.
Another thing to do is to assess whether the tools given to that person are appropriate. An indicator of inappropriate tool is how often end users ask for the raw data. Sometimes a dashboard is not the best tool to diagnose the root causes. The need is to do benchmarking, hypothesis testing, and A/B testing, all are better performed with Jupyter Notebooks, or raw excel files.
Obstacle 4: Turn insights into actions
While it may seem obvious that we need to take action based on data-driven insights, there are often cultural resistant, time constraints, or lack of resources that prevent actions to be taken.
Great data leaders incentivize people to take data-driven actions. They make it effortless for the insights to be delivered, via relevant forums, instant notifications or reverse-ETL. They make it easier for the action to be identified with prescriptive analysis (i.e data-enabled recommendation systems, often in a form of ML). They can even automate the actions, embed predictive and prescriptive analysis into production environment, like what Netflix did with their dynamic sizzles.
Enabling smart actions at scale, however, requires investment — investment that most data leaders will struggle to get. Without having a sound data strategy, quick-wins, and trust across the organizations, data leaders will not be given the appropriate investment to create break-through.
3. Measure the impact of data contribution
After the hard parts — identifying and contributing toward the right KPIs, it is time for the final step in delivering tangible ROI — measuring the impact.
Obstacle 5: Embed impact measurement into data practitioners’ jobs description
Some data teams don’t take the extra step to measure their impact. They are too occupied with shipping to production, or dealing with ad hoc requests. These teams still operate like a software team, or service team. They work based on release timeline, SLA, and stay far away from business initiatives, unaware of the results of their work. They are created that way, and therefore operate that way.
The lack of focus on impact measure has been built into the data organization itself. Looking up and down the organizational structure, data leaders cannot rely on the Data Engineer, Data Architect, DevOps Engineer, Data Privacy Officer, Data Governance Officer or Data Steward to focus on measuring the impact of data. It’s not the objective of their jobs. One may have some hope for the Data Product Manager, but the role is in its infancy, and the whole community is still debating what is actually a data product.
Someone has to lead the team to measure the impact, do the maths, and communicate that to the boardroom. Ideally, that person will be the CDO, or the most senior data leader in the organization. However, I think the data teams can benefit from a cultural change, where every data practitioner has ROI as the top-of-mind metric. Data teams should operate more like start-up incubators, where every project is an investment. All data practitioners should keep close eyes on the returns of their time, money and efforts.
Conclusion
Putting it all together, there are 5 fundamental obstacles standing in the way of delivering tangible data ROI:
Step 1: Have KPIs that represent “profitability” of data
Obstacle 1: Access the board room for the right business-facing KPIs
Step 2: Contribute to improving the KPIs
Obstacle 2: Set the right expectation for data
Obstacle 3: Identify what drive the KPIs
Obstacle 4: Turn insights into actions
Step 3: Measure the impact of data contribution
Obstacle 5: Embed impact measurement into data practitioners’ jobs description
They are simple, mutually exclusive and completely exhaustive. Depends on the circumstances at each organizations, data leaders may face each of these obstacles at different levels of severity. Nevertheless, by committing to resolve them one by one, data leaders will eventually harvest the fruits of their labor — tangible ROI!
For AI KPI management advice, connect with us at hello@itinai.com. And for continuous insights into leveraging AI, stay tuned on our Telegram t.me/itinainews or Twitter @itinaicom.
Spotlight on a Practical AI Solution
Consider the AI Sales Bot from itinai.com/aisalesbot designed to automate customer engagement 24/7 and manage interactions across all customer journey stages.
Discover how AI can redefine your sales processes and customer engagement. Explore solutions at itinai.com.