The article describes the author’s nostalgic reflection on a student project about crop yield and price prediction during their Master’s degree. They formed a team and chose a topic related to geographic information analysis and economics. The project involved data analysis, statistical modeling, and visualization, leading to successful outcomes and valuable lessons.
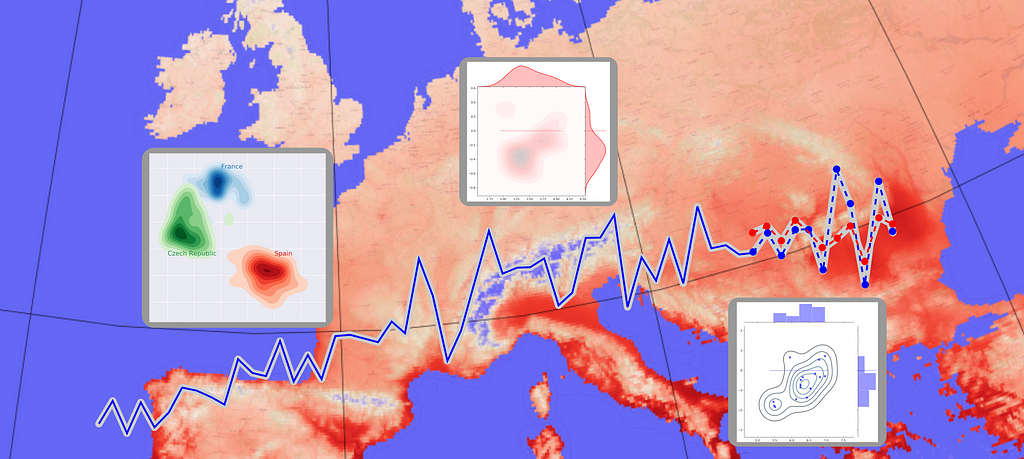
“`html
A Data Science Course Project About Crop Yield and Price Prediction I’m Still Not Ashamed Of
During the Christmas holidays, I experienced a feeling of nostalgia for the past student years. That’s why I decided to write a post about a student project that was done almost four years ago as a project on the course “Methods and models for Multivariate data analysis” during my Master’s degree in ITMO University.
Choosing the Topic
I suggested choosing a theme that was big enough that we could work independently on different parts of it and the domain which was close to our interests (geographic information analysis for me and economics for my colleagues).
Research & Data Sources
We started with a literature review to understand exactly how crop yield and crop price are predicted. We also wanted to understand what kind of forecast error could be considered satisfactory.
Climate Data Preprocessing
We have started with an assumption that wheat, rice, maize, and barley yields depend on weather conditions in the first half of the year. Thus, we obtained matrices for the whole territory of Europe with calculated features for the future model(s).
Aggregation of Information by Country
Not all of the country’s territory is suitable for agriculture. Therefore, it was necessary to aggregate information only from certain pixels. In order to account for the location of agricultural land, the following matrix was prepared.
Time Series Forecasting
Putting this method into practice proved to be the easiest. For example, in Python there are several libraries that allow to customize and apply the ARIMA model, for example pmdarima.
Ensembling
After all the models were built, we explored exactly how each model is “mistaken”. The Kalman filter was used to improve the quality of the forecast.
Futures Price Prediction
And the final part: model (lasso regression), which used predicted yield values and Futures features to estimate possible price values.
Why I Still Think This Project Is a Good One
So that’s the end of the story. Above there were posted some of tips. And in the last paragraph, I want to summarize the final point and say why I am satisfied with that project. Here are three main items:
Organisation of work and choice of topic, Meaningful theme, Hard skills. Well, we also got great marks on the exam XD
“`