Material scientists at the University of Rochester are using machine learning to expedite the discovery of new crystalline materials with specific properties. By automating the classification of materials based on X-ray diffraction patterns using convolutional neural networks, this approach aims to accelerate materials innovation and benefit various technological applications, from electronics to sustainability.
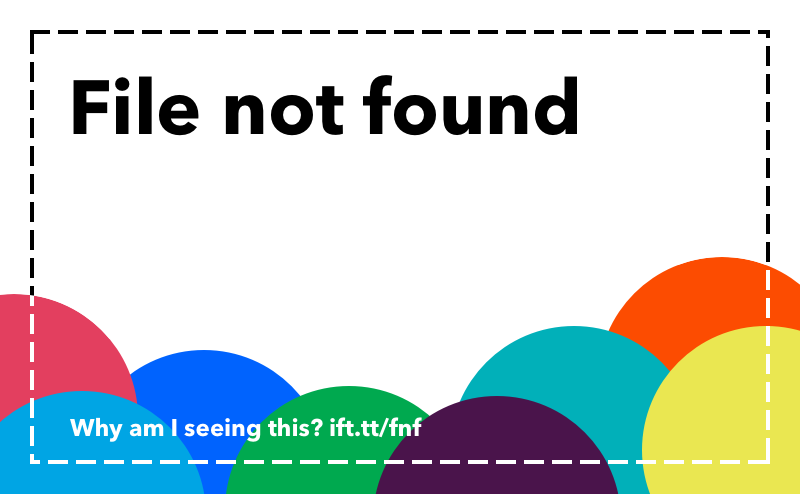
ML boosts X-ray diffraction techniques to find new materials
Material scientists at the University of Rochester are using machine learning to accelerate the discovery of new materials. By automating the analysis of X-ray diffraction (XRD) patterns, researchers are able to process the overwhelming amount of data produced, facilitating the development of materials with specific properties.
Automating material analysis
The study led by materials science PhD student Jerardo Salgado developed deep learning models utilizing convolutional neural networks (CNNs) to automate the classification of materials based on their XRD patterns. These models were trained on a large dataset of synthetic XRD patterns, allowing for the rapid analysis of material properties, which can significantly expedite materials innovation and development for various technological applications.
Research from the Center for Matter at Atomic Pressures also indicates that using XRD combined with machine learning can not only assist in discovering new materials but also provide insights into the formation of stars and planets.
By utilizing AI to automate data analysis, scientists can focus their efforts on designing materials that will shape the future, driving innovation and scientific discovery.
Using AI to redefine work processes
If you want to evolve your company with AI and stay competitive, consider using machine learning to revolutionize your materials discovery processes. AI solutions like the AI Sales Bot from itinai.com/aisalesbot can automate customer engagement and enhance interactions across all customer journey stages, redefining sales processes and customer engagement.
Practical AI implementation
For middle managers, it’s important to identify automation opportunities and define KPIs to ensure that AI endeavors have measurable impacts on business outcomes. Choosing tools that align with your needs and provide customization is essential. Implement AI gradually, starting with a pilot and expanding usage judiciously to maximize its benefits for the organization.
For AI KPI management advice and continuous insights into leveraging AI, connect with us at hello@itinai.com or stay tuned on our Telegram t.me/itinainews or Twitter @itinaicom.