This blog post outlines the capabilities of diffusion models for generating custom data by using additional conditioning. It introduces methods such as Stable Diffusion Inpainting, ControlNet, and GLIGEN, and highlights how fine-tuning with the Low-Rank Optimization technique, or LoRA, can efficiently adapt these methods to specific use cases. The article emphasizes the benefits of enhancing data diversity and quality for business projects.
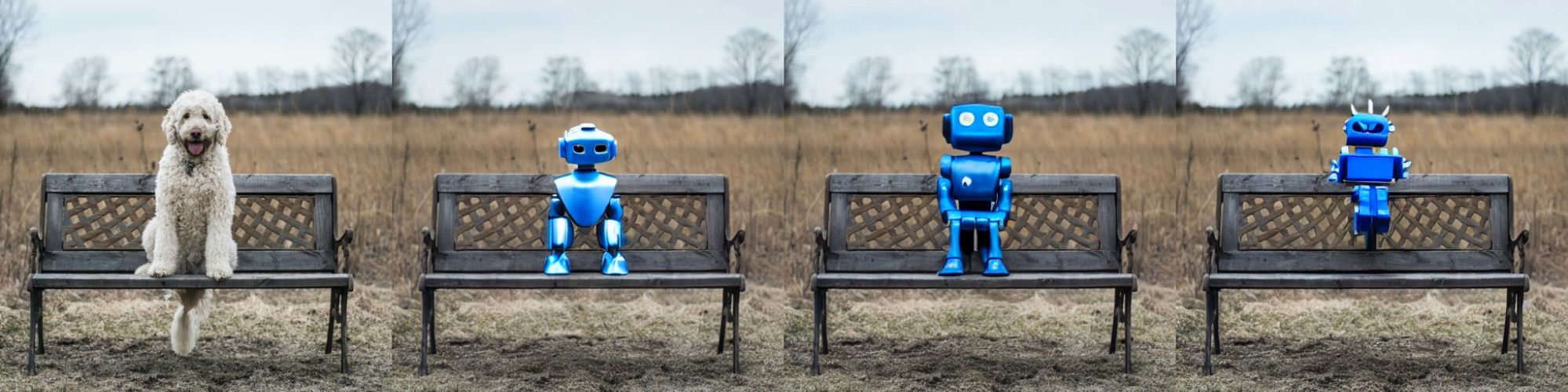
Generating Custom Data with Diffusion Models
Our recent work on data generation using diffusion models has led to exciting developments in the field. In this blog post, we will explore methods for generating images based on additional conditions and how these techniques can benefit real-world business projects.
Stable Diffusion Inpainting
The Stable Diffusion Inpainting method allows for the editing of specific parts of an image by providing a mask and a text prompt. This technique is useful for erasing and replacing parts of an image, and it relies on a modified UNet architecture to achieve this functionality.
ControlNet
ControlNet modifies diffusion models by adding a component ready to be trained with additional inputs. This approach enables the network to learn conditional control, directing outputs with segmentation masks, key points, edges, and more.
GLIGEN
GLIGEN introduces new layers called Gated Self-Attention (GSA) to process grounding input within the encoder. This method operates by processing a concatenation of inputs using the Transformer layer, making it a versatile choice for various conditions and visual features.
Training the Models
While pre-trained models are impressive, re-training is often necessary to meet the exact needs of business projects. However, training large diffusion models can be challenging due to the need for large datasets and significant computational power.
LoRA: Low-Rank Adaptation
LoRA is a fine-tuning method that enables swift and efficient learning of new styles or objects, even with limited data. This technique has exhibited remarkable flexibility and versatility in customizing diffusion models.
Results for Cityscapes
We have successfully demonstrated the effectiveness of the Stable Diffusion model with the LoRA method on the Cityscapes dataset, showcasing great fidelity in generating images that mimic the training dataset.
By leveraging these practical AI solutions, businesses can enhance data diversity and quality, opening up new possibilities for evolving with AI and staying competitive in the market.
For further insights into leveraging AI for your business, connect with us at hello@itinai.com or follow us on Telegram and Twitter.
Spotlight on a Practical AI Solution: AI Sales Bot
Consider the AI Sales Bot designed to automate customer engagement 24/7 and manage interactions across all customer journey stages. Explore solutions at itinai.com/aisalesbot.