Contemporary machine learning relies on foundation models (FMs), often utilizing sequence models, such as the Transformer, which has drawbacks concerning window length and description of material. A new family of models, structured state space sequence models, addresses these issues and has been shown effective in certain domains. Researchers have introduced Mamba, a novel SSM architecture, offering improved efficiency for multilingual deep learning applications. The architecture is capable of outperforming comparable Transformers in language modeling with linear scaling and higher inference throughput.
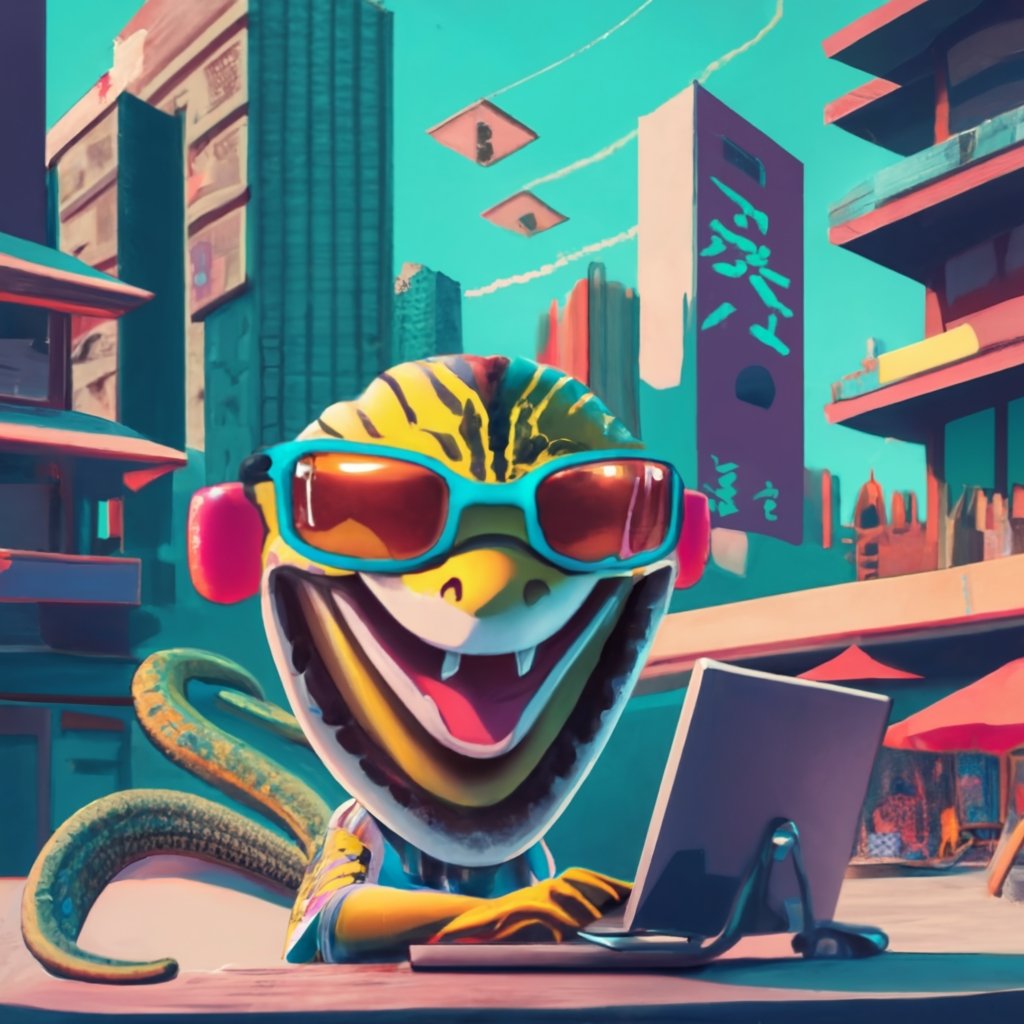
“`html
Contemporary Machine Learning and Practical Solutions
Foundation Models and Sequence Models
Contemporary machine learning utilizes foundation models (FMs) which are vast models pre-trained on large amounts of data and then modified for specific tasks. These models commonly use sequence models, operating on various input types such as language, pictures, voice, audio, time series, and genomes. The Transformer and its central attention layer are foundational in contemporary FMs, enabling effective representation of complex information.
Challenges and Structured State Space Models
However, these models face challenges with scaling and long-range context. To address these issues, structured state space models offer a promising solution. These models exhibit linear or almost linear scaling in sequence length and have shown effectiveness in certain data modalities like audio and vision.
Innovation in State Space Models
A research team from Carnegie Mellon University and Princeton University has proposed a novel category of state space models, enhancing the Transformer-like modeling capability while maintaining a linear relationship with sequence length.
Introducing Mamba: A Breakthrough in Sequence Modeling
Mamba Architecture Features
Mamba incorporates selective state space models and offers high quality, fast inference and training, and long context capabilities. This architecture serves as the cornerstone for broader foundation models operating on sequences, and has shown promising performance across various data modalities and tasks.
Applications and Performance
Mamba outperforms previous state-of-the-art models in tasks like modeling audio waveforms, DNA sequences, and language processing. It demonstrates superior performance and faster generation throughput, making it a compelling option for language models and other deep learning applications.
Connect with Us
For insights into leveraging AI and practical AI solutions, connect with us at hello@itinai.com, or stay tuned on our Telegram or Twitter.
Practical AI Solution: AI Sales Bot
Consider the AI Sales Bot designed to automate customer engagement 24/7 and manage interactions across all customer journey stages. Explore more at itinai.com/aisalesbot.
Evolve with AI
Discover how AI can redefine your company’s operations and customer engagement, revolutionizing your sales processes. Find out more at itinai.com.
“`