AWS’s suite of low-code and no-code ML tools, such as Amazon SageMaker Canvas, enables rapid, cost-effective machine learning model development without requiring coding expertise. Deloitte uses these tools to expedite project delivery and take on more clients, increasing accessibility and standardization while reducing time and costs, resulting in roughly 30-40% productivity gains in ML development and deployment.
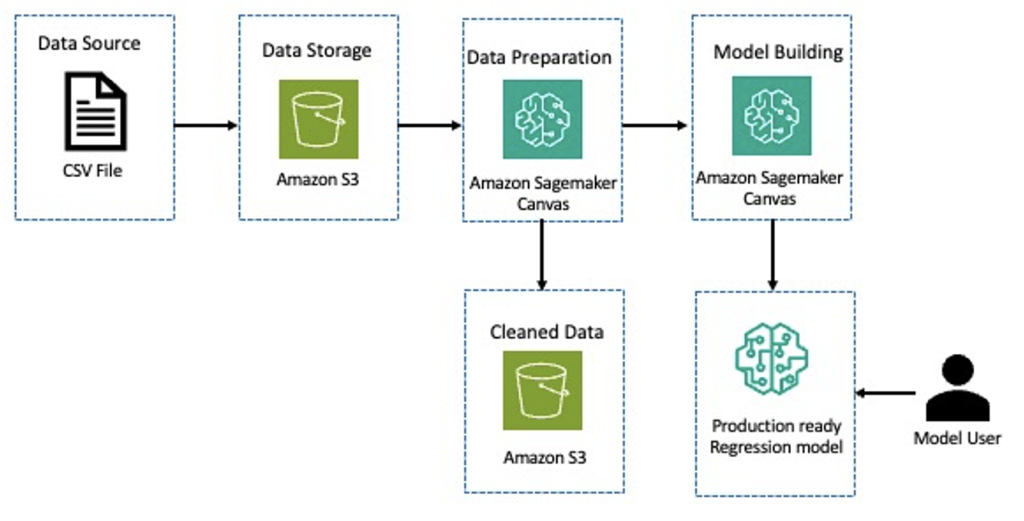
“`html
Streamline Machine Learning with AWS No-Code Tools
Machine Learning (ML) development can be a complex and time-consuming process, often taking months and requiring specialized expertise. But with AWS’s low-code and no-code ML services, such as Amazon SageMaker Canvas, you can build and deploy ML models quickly and without writing code, making ML accessible to everyone.
Why Deloitte Chooses AWS No-Code ML Tools:
- Accessibility: Non-programmers can now build ML models with ease.
- Rapid Technology Adoption: Stay ahead with the latest ML technology without the hassle.
- Cost-Effective Development: Save time and money while achieving a higher return on investment.
Benefits of Using SageMaker Canvas:
- Faster Data Preparation: Over 300 built-in transformations and natural language capabilities speed up data readiness.
- Efficient Model Building: Use ready-to-use models or AutoML for quick custom model creation.
- Easier Deployment: Deploy models in just a few clicks with SageMaker endpoints.
Vishveshwara Vasa, Cloud CTO for Deloitte, highlights a 30–40% increase in development and deployment productivity by using these tools.
Building a Loan Default Prediction Model with SageMaker Canvas:
Follow a no-code approach to predict loan defaults, helping financial services manage risk and gain a competitive edge. SageMaker Canvas simplifies the entire ML workflow from data preparation to deployment on a single platform.
Key Steps in Data Preparation and Model Building:
- Interactive data exploration and transformation without coding.
- Use natural language to apply feature engineering transformations.
- Export cleansed data and features for model development.
Deploying Your ML Model:
After building your model, deploy it easily for real-time predictions and manage risk more effectively.
Clean Up:
Remember to delete any resources you’re no longer using to avoid additional charges.
Conclusion
No-code ML by AWS offers a faster, simpler, and more cost-effective approach to ML development. Deloitte’s use of these tools has significantly reduced their ML model build timelines.
About the Authors:
Chida Sadayappan, Kuldeep Singh, and Kasi Muthu are experts at Deloitte and AWS, respectively, with a passion for driving AI/ML innovation.
Take Your Company Forward with AI:
Boost productivity and stay competitive with no-code/low-code ML solutions. For more information, reach out to hello@itinai.com and follow us on Telegram and Twitter for the latest AI insights.
Spotlight on Practical AI:
Explore the AI Sales Bot for automating customer engagement around the clock. Learn more at itinai.com/aisalesbot.
“`