This research paper introduces a novel deep learning model to address the challenge of understanding alternative splicing in genes. The model combines sequence information, structural features, and wobble pair indicators to accurately predict splicing outcomes. Its interpretability, achieved through a carefully designed training process and the Tuner function, sets it apart from traditional methods. Researchers can use this tool to explore the intricate world of alternative splicing and uncover the mechanisms that govern gene regulation.
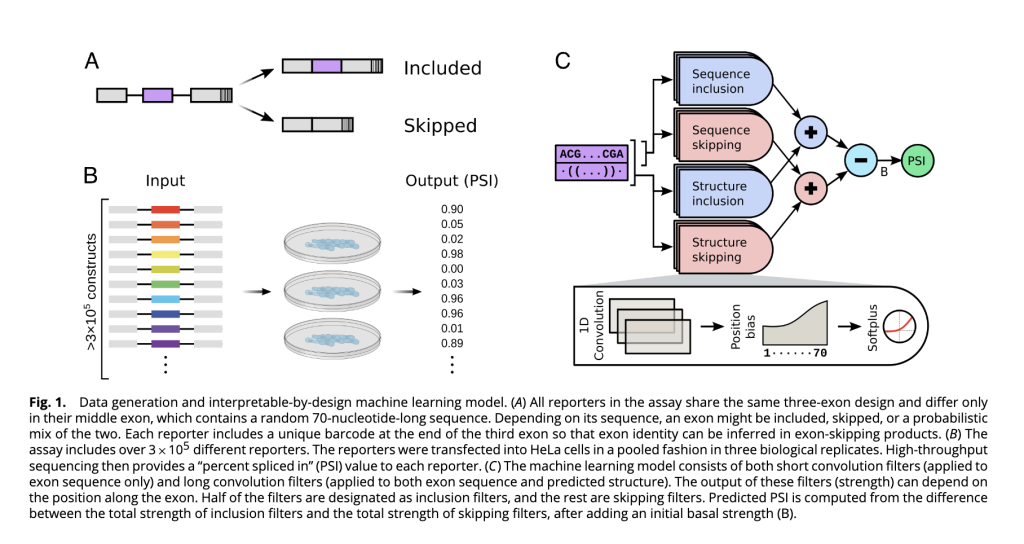
Unraveling Gene Regulation with Deep Learning: A New AI Approach to Understanding Alternative Splicing
Alternative splicing is a crucial process in gene regulation, allowing a single gene to produce multiple mRNA variants and different protein isoforms. However, analyzing the complex patterns of alternative splicing has been a challenge for scientists. In a recent research paper, a team of researchers introduces a novel deep learning model to address this challenge and provide insights into alternative splicing regulation.
An Efficient and Accurate Approach
Traditional methods used to study alternative splicing in gene regulation can be time-consuming and limited when analyzing vast amounts of genomic data. The researchers recognized the need for a more efficient and accurate approach. They developed a cutting-edge deep learning model that leverages neural networks to predict splicing outcomes, making it a valuable tool for researchers.
The model operates in a multi-step training process, progressively incorporating learnable parameters to enhance interpretability. It integrates diverse sources of information, including sequence and structural data. The model uses strength-computation modules (SCMs) to compute the strengths associated with different splicing outcomes, capturing important sequence motifs through convolutional layers. It also considers structural features by using dot-bracket notation to capture complex secondary structures that can influence splicing decisions.
The model’s distinguishing feature is the Tuner function, a learned nonlinear activation function. It maps the difference between strengths associated with inclusion and skipping splicing events to a probability score, predicting the percentage of spliced-in (PSI) values. This prediction allows researchers to understand how alternative splicing may be regulated in specific contexts.
Proven Performance and Reliability
The researchers rigorously evaluated the model’s performance using various assays and datasets. By comparing its predictions to experimental results, they demonstrated its ability to accurately identify essential splicing features. The model effectively distinguishes between genuine splicing features and potential artifacts, ensuring the reliability of its predictions.
A Comprehensive Solution for Understanding Alternative Splicing
This groundbreaking research paper presents a comprehensive solution for understanding alternative splicing in genes. The deep learning model combines sequence information, structural features, and wobble pair indicators to accurately predict splicing outcomes. Its interpretability, achieved through a carefully designed training process and the Tuner function, sets it apart from traditional methods.
Researchers can use this model to explore the intricate world of alternative splicing and uncover the mechanisms that govern gene regulation.
Implementing AI in Your Company
Check out the original research paper to learn more about this innovative approach to alternative splicing.
If you want to evolve your company with AI and stay competitive, consider implementing AI solutions like the one discussed in this research paper. Here are some practical steps to follow:
- Identify Automation Opportunities: Locate key customer interaction points in your business that can benefit from AI.
- Define KPIs: Ensure that your AI initiatives have measurable impacts on business outcomes.
- Select an AI Solution: Choose tools that align with your needs and can be customized to fit your specific requirements.
- Implement Gradually: Start with a pilot project, gather data, and expand the use of AI judiciously.
For AI KPI management advice and insights into leveraging AI, connect with us at hello@itinai.com. Stay updated on the latest AI research news and projects by joining our ML SubReddit, Facebook Community, Discord Channel, and subscribing to our Email Newsletter.
If you’re interested in AI-driven sales automation, check out the AI Sales Bot from itinai.com/aisalesbot. It is designed to automate customer engagement and manage interactions throughout the customer journey stages.
Discover how AI can redefine your sales processes and customer engagement. Explore solutions at itinai.com.